현재 많은 기업들이 AI 도입에 열을 올리고 있지만, 과연 이러한 기술 도입이 실질적인 임팩트를 창출하고 있는지에 대한 본질적인 질문이 필요한 시점입니다. AI 기술은 단순히 도입하는 것만으로는 의미가 없으며, 조직과 고객에게 실질적인 가치를 제공할 때만 그 의미가 있습니다. 이 글에서는 조직의 성공적인 AI 도입을 위한 핵심 전략과 실제 임팩트 창출 방안에 대해 살펴보겠습니다.
AI 도입, 진짜 필요한가? 우리 조직에 ‘임팩트’가 없다면 무의미하다! (조용민 대표 2편)
이 영상은 AI 도입에 대한 **본질적인 질문**을 던지며, 조직에 실질적인 '임팩트'가 없다면 AI 도입은 무의미하다고 강조합니다. AI 에이전트 기술이 일상을 문제로 인식하고 개선하는 역량에 초
lilys.ai
AI 도입의 필요성과 에이전트 경쟁 구도의 이해
현재 AI 기술의 급속한 발전으로 인해 산업 전반의 업무 환경이 크게 변화하고 있습니다. 특히 주목할 만한 점은 주니어 개발자의 역할과 필요 역량이 변화하고 있다는 것입니다. 과거에는 코딩 능력이 주니어 개발자의 핵심 역량이었다면, 이제는 문제 발견 및 개선 능력이 더욱 중요해지고 있습니다.
AI의 발전은 센트럴라이즈된 형태로 강력해지고 있으며, 새로운 유니크한 서비스들이 지속적으로 등장하고 있습니다. 그러나 안타깝게도 이러한 새로운 서비스들은 결국 기존의 강력한 플랫폼에 흡수될 위험이 큽니다. 이는 마치 앱 생태계가 구글과 애플의 플랫폼에 종속된 것과 유사한 현상이라고 볼 수 있습니다.
현대 AI 경쟁에서 가장 중요한 요소는 더 편리한 사용자 경험을 제공하는 것입니다. 서비스 발화 액션의 출처가 무엇인지보다 사용자가 얼마나 쉽고 편리하게 서비스를 이용할 수 있는지가 모든 경쟁의 핵심이 될 것입니다.
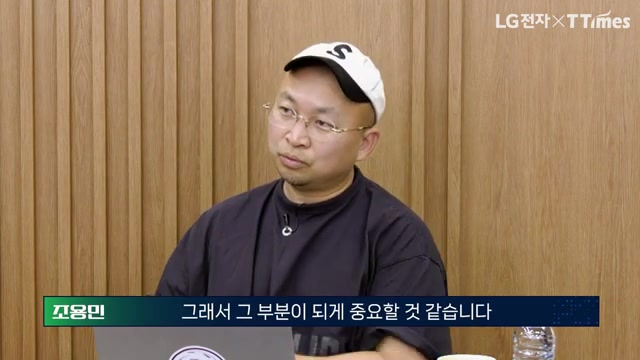
AI 기술과 사용자 이익의 중요성
AI 기술 도입에 있어 가장 중요한 것은 사용자에게 명확한 혜택을 제공하는 것입니다. 아무리 뛰어난 AI 기술이라도 사용자에게 실질적인 가치를 제공하지 못한다면 오래 살아남지 못할 것입니다.
최근 주니어 개발자 지원이 급증한 현상은 시장에서의 필요가 변화했음을 보여줍니다. AI가 많은 기본적인 코딩 작업을 수행할 수 있게 되면서, 단순 코딩 능력보다는 문제를 해결하는 능력이 더욱 중요해졌습니다. 이에 따라 교육 방식도 변화가 필요하며, 젊은 개발자들이 새로운 형태의 역량을 갖출 수 있도록 교육 시스템이 개선되어야 합니다.
모바일 시대와 마찬가지로, AI 에이전트 시대에서도 궁극적으로 고객의 니즈를 충족시키는 서비스가 성공할 것입니다. 사용자의 불편함을 최소화하고 명확한 베네핏을 제공하는 AI 서비스만이 시장에서 살아남을 수 있을 것입니다.
AI의 필요성과 사용자 중심 서비스의 중요성
실제 성공 사례를 살펴보면, 사용자 중심의 서비스를 제공하는 기업들이 높은 성과를 거두고 있습니다. 어떤 플랫폼은 4,800만 명의 사용자를 확보했는데, 이는 사용자 친화적인 노력이 사용자에게 긍정적인 반응을 이끌어냈기 때문입니다.
성공적인 기업들의 공통점은 단순히 데이터를 수집하는 데 그치지 않고, 데이터 속 패턴을 읽고 해석할 수 있는 능력을 갖추고 있다는 점입니다. 이를 통해 사용자 중심의 서비스를 제공하는 것이 비즈니스 성공의 핵심입니다.
실제 미국 로스앤젤레스의 병원들은 의사들이 업무 중 키보드 사용을 최소화하고 대화형 인터페이스를 통해 진료를 진행하도록 하여 환자 서비스의 질을 크게 향상시켰습니다. 이는 기술 도입의 목적이 사용자 경험 개선에 맞춰졌기 때문에 가능했던 성과입니다.
반면, 아마존고의 실패 사례는 사용자의 실제 필요를 제대로 파악하지 못하고 단순히 결제 편리성만을 강조한 것에 기인합니다. 이와 대조적으로 에레혼 슈퍼마켓은 다양한 상품을 시각적으로 노출시키고 고객과의 소통을 강화함으로써 더 나은 쇼핑 경험을 제공하여 성공을 거두었습니다.
AI 도입의 효과와 사용자 중심의 접근 방식
AI 도입에서 가장 중요한 것은 유저 베네핏을 우선적으로 고려하는 것입니다. 이러한 접근 방식을 취하는 조직이 시장에서 성공할 가능성이 높습니다. 따라서 조직의 비즈니스 모델도 고객의 욕구를 깊이 이해하고 새로운 가치를 제공하는 방향으로 발전해야 합니다.
성공적인 AI 도입을 위해서는 단순한 데이터 수집을 넘어 사용자의 숨겨진 니즈와 패턴을 읽어내는 능력이 필수적입니다. 예를 들어, 건물의 균열 패턴을 분석하여 문제가 발생하기 전에 예방 조치를 취하는 것처럼, 고객 데이터의 패턴을 분석하여 미래의 니즈를 예측하고 대응하는 것이 중요합니다.
AI는 비즈니스 문제 해결의 중요한 도구로 떠오르고 있으며, 데이터 기반의 의사결정을 통해 조직의 성과를 크게 향상시킬 수 있습니다. 이 과정에서 창의적인 접근 방식이 필수적이며, 이를 통해 기존에는 발견하지 못했던 새로운 비즈니스 기회를 창출할 수 있습니다.
인재 관리 측면에서도 변화가 필요합니다. HR 부서는 단순한 인사 관리를 넘어 비즈니스 문제를 해결하는 방향으로 역할을 재정의해야 합니다. 이는 AI 시대에 맞는 새로운 인재 관리 패러다임으로, 조직의 성과 향상에 직접적으로 기여할 수 있습니다.
AI 도입의 필요성과 교육 방향성
AI 도입은 단순히 기술 자체가 목적이 아니라 비즈니스 문제 해결을 목표로 해야 합니다. 이를 위해 AI 교육은 현업의 실제 문제를 중심으로 구성되어야 하며, 구성원들이 직접 문제를 풀어보는 실습 중심의 교육이 효과적입니다.
교육 담당자는 AI 활용 방안을 심도 있게 고민하여 교육 내용을 마련해야 하며, 교육 종료 후에는 구성원들의 AI 리터러시 향상 정도를 객관적으로 평가할 필요가 있습니다. 성과 중심의 AI 교육이 이루어져야 실질적인 비즈니스 임팩트를 창출할 수 있습니다.
HR 담당자는 단순히 트렌드를 따라가는 불필요한 교육을 지양하고, 조직의 실제 필요에 맞는 교육을 제공해야 합니다. 무분별한 AI 교육은 오히려 구성원들의 피로도만 증가시킬 뿐, 실질적인 효과를 거두기 어렵습니다.
리더는 경영 전반에 대한 비즈니스 임팩트를 이해하고, 조직 구성원들의 미래 필요성을 바탕으로 명확한 교육 목적을 설정해야 합니다. HR의 궁극적인 미션은 비즈니스 임팩트를 창출하는 것이며, 이를 위해 교육 프레임워크와 평가 방법론을 재정립해야 합니다.
AI 시대의 생존 전략: 고객 중심의 가치 창출
AI 시대에 성공하기 위한 핵심은 고객의 니즈를 깊이 이해하고 이를 기반으로 새로운 가치를 창출하는 것입니다. 실질적인 임팩트가 없는 AI 도입은 단순한 비용 낭비에 그칠 뿐이므로, 조직은 AI 기술이 어떻게 고객과 비즈니스에 실질적인 가치를 제공할 수 있는지에 초점을 맞춰야 합니다.
AI가 발전할수록 역설적으로 인간적인 요소가 더욱 중요해지고 있습니다. 데이터를 수집하고 분석하는 것을 넘어, 그 속에서 의미 있는 패턴을 발견하고 창의적인 해결책을 제시할 수 있는 능력이 미래 경쟁력의 핵심이 될 것입니다.
결국 AI 시대의 승자는 기술 자체가 아닌, 기술을 활용하여 고객에게 새로운 가치를 제공할 수 있는 기업이 될 것입니다. 임팩트 없는 AI 도입은 의미가 없으며, 진정한 성공은 기술을 통해 고객과 사회에 실질적인 가치를 창출할 때 이루어질 것입니다.
AI Implementation Without Real Impact is Meaningless: Key Strategies for Successful AI Transformation in Organizations
While many companies are rushing to adopt AI, it's time to ask the fundamental question: is this technology adoption creating real impact? AI technology has no meaning simply by being implemented; it only becomes meaningful when it provides real value to organizations and customers. In this article, we'll explore key strategies for successful AI adoption and ways to create actual impact.
Understanding the Necessity of AI Adoption and the Agent Competition Landscape
The rapid development of AI technology is dramatically changing work environments across industries. Notably, the role and required capabilities of junior developers are evolving. If coding ability was once the core competency of junior developers, problem discovery and improvement skills are now becoming more important.
AI development is becoming increasingly centralized and powerful, with new unique services continually emerging. Unfortunately, these new services risk being absorbed by existing powerful platforms. This phenomenon is similar to how the app ecosystem has become dependent on Google and Apple platforms.
In modern AI competition, the most critical factor is providing a more convenient user experience. Rather than focusing on the source of service activation, what matters most is how easily and conveniently users can access services.
The Importance of AI Technology and User Benefits
The most important aspect of AI technology adoption is providing clear benefits to users. No matter how excellent an AI technology might be, it won't survive long if it doesn't deliver real value to users.
The recent surge in junior developer applications demonstrates changing market needs. As AI can perform many basic coding tasks, problem-solving ability has become more important than simple coding skills. Consequently, educational systems need to change, helping young developers acquire new types of competencies.
Just as in the mobile era, services that ultimately meet customer needs will succeed in the AI agent era. Only AI services that minimize user inconvenience and provide clear benefits will survive in the market.
The Necessity of AI and the Importance of User-Centered Services
Looking at successful cases, companies providing user-centered services are achieving high performance. One platform secured 48 million users because user-friendly efforts elicited positive responses from users.
A common trait among successful companies is not just collecting data but having the ability to read and interpret patterns in data. Providing user-centered services based on this capability is key to business success.
In Los Angeles hospitals, doctors have minimized keyboard use during work and conduct consultations through conversational interfaces, significantly improving patient service quality. This achievement was possible because the purpose of technology adoption was focused on improving user experience.
Conversely, Amazon Go's failure stemmed from not properly understanding users' actual needs and simply emphasizing payment convenience. In contrast, Erewhon Supermarket achieved success by visually exposing various products and strengthening communication with customers to provide a better shopping experience.
The Effects of AI Adoption and User-Centered Approach
The most important aspect of AI adoption is prioritizing user benefits. Organizations taking this approach are more likely to succeed in the market. Therefore, organizational business models should evolve to deeply understand customer desires and provide new value.
For successful AI adoption, it's essential to go beyond simple data collection and develop the ability to read users' hidden needs and patterns. For example, like analyzing building crack patterns to take preventive measures before problems occur, it's important to analyze customer data patterns to predict and respond to future needs.
AI is emerging as an important tool for solving business problems and can greatly enhance organizational performance through data-driven decision-making. In this process, creative approaches are essential, potentially creating new business opportunities previously undiscovered.
Talent management also needs to change. HR departments must redefine their role toward solving business problems beyond simple personnel management. This represents a new talent management paradigm suited to the AI era, directly contributing to organizational performance improvement.
The Need for AI Adoption and Educational Direction
AI adoption should aim at solving business problems rather than focusing on the technology itself. To this end, AI education should be centered on actual workplace problems, with effective learning through practice where members directly solve problems.
Education coordinators should deeply consider AI utilization methods to prepare educational content and objectively evaluate members' AI literacy improvement after training. Performance-centered AI education is necessary to create substantial business impact.
HR managers should avoid unnecessary training that simply follows trends and provide education that meets the organization's actual needs. Indiscriminate AI training only increases member fatigue without achieving tangible results.
Leaders must understand business impact across management and establish clear educational objectives based on future needs of organizational members. HR's ultimate mission is to create business impact, requiring redefinition of educational frameworks and evaluation methodologies.
Survival Strategy in the AI Era: Customer-Centered Value Creation
The key to success in the AI era is deeply understanding customer needs and creating new value based on them. AI adoption without substantial impact is merely a waste of costs, so organizations should focus on how AI technology can provide real value to customers and business.
Paradoxically, as AI advances, human elements become increasingly important. Beyond collecting and analyzing data, the ability to discover meaningful patterns and present creative solutions will be central to future competitiveness.
Ultimately, the winners in the AI era will not be technology itself but companies that can provide new value to customers using technology. AI adoption without impact is meaningless, and true success will come when technology creates substantial value for customers and society.
태그: #AI도입, #인공지능전략, #비즈니스임팩트, #AI에이전트, #사용자경험, #고객중심, #데이터분석, #AI교육, #HR전략, #비즈니스혁신, #디지털전환, #기술도입, #가치창출, #조직혁신, #미래경쟁력
Citations:
- https://openreview.net/pdf/f544a00e48924bb0e4cc615ce8730c7964786a18.pdf
- https://openreview.net/pdf/313df058e10cbae68e611612d244e3bbaed8d568.pdf
- https://openreview.net/pdf?id=cC61W9tsD3U
- https://arxiv.org/abs/2308.00868
- https://arxiv.org/html/2412.04862v1
- https://arxiv.org/pdf/2501.04410.pdf
- https://arxiv.org/html/2502.05651v1
- https://arxiv.org/html/2403.06537v2
- https://openreview.net/pdf/0ac061fee1c25d1a3f666686e498ea96e1d351f8.pdf
- https://www.semanticscholar.org/paper/25cac745a234f63754dedcf3809e6f6bf0a424e9
- https://www.semanticscholar.org/paper/b86f9583335479a62d965a3f0268c3725e07b006
- https://www.semanticscholar.org/paper/cc8b8824757b3109676d05f33a70741b1db31fbb
- https://www.semanticscholar.org/paper/31b59b9069c693441575f234eb46ce5075ade0d0
- https://www.semanticscholar.org/paper/1cc6d004de2a5237bb5743272625b9292428a20e
- https://www.semanticscholar.org/paper/4effcc73048d23331cd256a5fb5e5b0b19ce3a90
- https://www.semanticscholar.org/paper/94905c151f7e09f157127a4e385b1c5fffd4476f
- https://www.semanticscholar.org/paper/f3c6d63582774171e610a313d974d26d2c06674d
- https://www.semanticscholar.org/paper/888ef2804d9a18e7f7d1d2197209eedde8d86db9
- https://www.semanticscholar.org/paper/f2d522a13c51244de7ac4ee9553efa0553190271
- https://www.semanticscholar.org/paper/da7c4980300b243ff6bbf4762a3b3624e72d030d
- https://arxiv.org/html/2410.00683v1
- https://arxiv.org/abs/2204.11642
- https://arxiv.org/pdf/2403.00137.pdf
- https://openreview.net/pdf/2cf7397307d6674003c680a8f10d256f83e05d22.pdf
- https://arxiv.org/abs/2309.12482
- https://arxiv.org/abs/2304.10578
- https://arxiv.org/abs/2412.19530
- https://arxiv.org/html/2502.20243v1
- https://arxiv.org/abs/1812.10404
- https://arxiv.org/abs/2307.10265
- https://www.semanticscholar.org/paper/d8a8fe66a4b4b2e63be3cb96b3dae01836c4d0d6
- https://www.semanticscholar.org/paper/1dfdd9b37e8336d5db8f79c213173754bbe938c6
- https://www.semanticscholar.org/paper/38d7fd51003209d59868d3e59abd62e29e757cce
- https://www.semanticscholar.org/paper/19d7cae17cfcef7fea6acc178f389581b5d63824
- https://www.semanticscholar.org/paper/4f08bb09badd04822b14fb91d870820a1618194b
- https://www.semanticscholar.org/paper/6ed45586c370400cfaa11a7757e1184657575cd4
- https://www.semanticscholar.org/paper/a4f2f72bbe89500c2e8511d5f59e27edca8cfc22
- https://www.semanticscholar.org/paper/95d350e68075152b6e5f3aee8c7d3a7dc4815a46
- https://www.semanticscholar.org/paper/0635ce6159c499c8867eb425bc002c96cc75111f
- https://www.semanticscholar.org/paper/5fa71ce59b9fac7ec37ec3e0716aed472e841db9
- https://www.semanticscholar.org/paper/e230019924826a623390059a58970f74e335cc2c
- https://www.semanticscholar.org/paper/98961a688cc21897199acedc0c2b593c312776b4
- https://www.semanticscholar.org/paper/e64365d0846476e06beeaa18626f356c967a6f5d
- https://www.semanticscholar.org/paper/79d9a3eb815cc6e537fcc62c651cf33cd3032cf4
- https://www.semanticscholar.org/paper/be7c9e7982c5a19cf1834c708197c7909d5e8608
- https://www.semanticscholar.org/paper/c153ca35d895930bcbc881a7b32ccda3c6937e6a
- https://www.semanticscholar.org/paper/ffcbeacfbfc76c9b313253e72a0fe556cca2fb45
- https://www.semanticscholar.org/paper/942258c66cd30b152fd486bbc1e128b242247eb3
- https://www.semanticscholar.org/paper/3d3ecaa76b8b971c55d4eb78399dfc205dff7915
- https://www.semanticscholar.org/paper/7c9a56c18e8fedd17277c2368d583a8a20141755
- https://www.semanticscholar.org/paper/a69a26900a14972e0404472e934bd1b0d701b966
'Agentic AI' 카테고리의 다른 글
AI 에이전트와 사용자 경험(UX): 성공적인 상호작용을 위한 디자인의 중요성 (0) | 2025.03.23 |
---|---|
PydanticAI로 구현하는 차세대 에이전틱 AI 시스템: 구조화된 데이터 처리의 새로운 지평 (0) | 2025.03.22 |
AI와 UX의 완벽한 조화: 기술이 아닌 사용자 중심 혁신을 말하다 (1) | 2025.03.22 |
미래를 바꿀 AI 에이전트 기술, 어디까지 왔나? (Anthropic의 Claude와 Manus AI 협업 사례) (1) | 2025.03.22 |
에이전트 오케스트레이션의 새 시대: Adobe의 AI 혁신이 가져올 미래 (0) | 2025.03.20 |