AI 에이전트 기술이 급속도로 발전하고 있는 가운데, 기업들은 2025년을 'AI 에이전트의 해'로 주목하고 있습니다. 코딩, 고객 지원부터 물리적 환경에서의 적용까지, AI 에이전트의 현재 모습과 미래 가능성을 살펴보며 기술 발전의 핵심을 파악해 봅니다.
AI Agents Still Struggling
이 영상은 **AI 에이전트** 기술이 현재 직면한 과제와 미래 가능성에 대해 논의합니다. 현재 AI 에이전트는 코딩, 고객 지원, 영업 분야에서 **일부 성과**를 보이고 있지만, 복잡한 시스템 통합과
lilys.ai
AI 에이전트의 현재: 성과와 한계점
오늘날 AI 에이전트 기술은 눈부신 발전을 이루고 있습니다. 여기서 AI 에이전트란 무엇일까요? 엔지니어링 관점에서 AI 에이전트는 자율 시스템 또는 대규모 언어 모델(LLM)이 스스로 행동을 결정하고 수행하는 형태로 이해됩니다. 현재 기업들은 AI 인프라 구축 단계를 넘어 실질적인 AI 응용 프로그램 개발에 집중하는 추세입니다.
기업 환경에서의 적용 사례
AI 에이전트 기술은 특히 세 가지 핵심 영역에서 두각을 나타내고 있습니다:
- 코딩 지원: 개발자들의 생산성을 향상시키는 코드 자동 생성 및 최적화
- 고객 지원: 24시간 대응 가능한 지능형 고객 응대 시스템 구축
- 영업 지원: 데이터 기반 인사이트를 통한 영업 프로세스 개선
실제 사례를 살펴보면, Maven AGI와 같은 기업은 AI 에이전트를 통해 기존 시스템과의 원활한 통합을 이뤄내며 시간과 비용을 크게 절약하는 동시에 실질적인 비즈니스 가치를 창출하고 있습니다. 이는 단순한 기술 도입이 아닌, 기업 전체 프로세스의 혁신을 의미합니다.
AI 에이전트 기술의 현실적 한계
하지만 눈부신 발전에도 불구하고, AI 에이전트 기술은 여전히 중요한 한계에 직면해 있습니다:
- 검증 가능성의 제한: 텍스트와 이미지 분야에서는 높은 반복성과 검증 가능성을 보이지만, 물리적 환경에서는 아직 제한적입니다.
- 워크플로우 통합의 어려움: 로봇 공학, 창고 관리와 같은 비공식적 데이터 접근이 필요한 분야에서는 통합이 어렵습니다.
- ROI 실현의 지연: 데이터와 워크플로우 통합은 발전 중이지만, 기업에서 실질적인 투자 수익을 얻기까지는 시간이 더 필요합니다5.
Daniel Pelling이 제시한 '물리적 AI' 이론에 따르면, 실제 환경에서의 AI 에이전트 적용은 보상 메커니즘의 구축이 필수적입니다. 그러나 이러한 보상 체계를 현실 세계에 구현하는 것은 디지털 환경보다 훨씬 복잡합니다.
소비자 경험의 복잡성: AI 에이전트의 실생활 적용
AI 에이전트가 비즈니스 환경을 넘어 일반 소비자의 삶에 들어왔을 때 발생하는 문제는 더욱 복잡합니다. 간단해 보이는 작업조차 실제로는 수많은 변수와 선택지를 고려해야 합니다.
항공편 예약: 복잡성의 좋은 예시
예를 들어, 항공편을 예약하는 간단한 작업을 생각해 봅시다. 이 과정에서 AI 에이전트는:
- 적절한 항공사 선택
- 최적의 공항과 경유지 파악
- 개인 선호에 맞는 시간대 선정
- 가격과 편의성 사이의 균형 맞추기
- 좌석 유형, 수하물 옵션, 마일리지 적용 등 세부사항 결정
이 모든 결정을 완벽하게 처리해야 만족스러운 소비자 경험이 가능합니다. 문제는 AI 에이전트가 복합적인 시스템으로, 작은 오류가 50단계에 걸쳐 누적될 경우 결과적으로 사용자 경험이 크게 저하될 수 있다는 점입니다.
오류 누적의 문제
AI 에이전트의 가장 큰 도전 중 하나는 오류 누적 문제입니다. 각 단계에서 95%의 정확도를 가진 시스템이 50단계를 거치면, 최종 결과물의 정확도는 크게 떨어질 수 있습니다. 이러한 문제는 특히 실시간 결정이 필요한 환경에서 더욱 두드러집니다.
"AI 에이전트 시스템의 진정한 가치는 개별 작업의 정확도가 아닌, 전체 프로세스를 얼마나 매끄럽게 완료하느냐에 달려 있습니다."
대기업들이 시장을 지배하는 상황에서도, 소규모 기업과 스타트업이 이러한 문제에 대한 혁신적인 해법을 제시할 기회가 있습니다. 대기업과의 전략적 협력을 통해 틈새 시장을 공략하는 것이 유망한 접근법입니다.
AI 인프라의 발전: 클라우드 플랫폼과 오픈소스의 역할
AI 에이전트 기술의 미래는 강력한 인프라에 달려 있습니다. 특히 클라우드 컴퓨팅 기술은 AI 모델을 훈련, 배포 및 확장하는 데 필요한 인프라를 제공함으로써 AI 에이전트 개발을 가속화하고 있습니다5.
클라우드 기반 AI 개발 환경의 중요성
Amazon Web Services(AWS), Microsoft Azure, Google Cloud와 같은 주요 클라우드 제공업체들은 생성형 AI를 위한 다양한 서비스를 제공하며, 이는 기업의 AI 에이전트 개발 속도를 크게 향상시키고 있습니다. 이러한 플랫폼은:
- 고성능 컴퓨팅(HPC) 리소스 제공
- 서버리스 아키텍처를 통한 유연한 확장
- 엣지 컴퓨팅을 통한 실시간 처리
- AI 특화 스토리지 솔루션
등의 기능을 제공하며 AI 에이전트 개발의 핵심 토대가 되고 있습니다5.
오픈소스 생태계의 양면성
오픈소스는 AI 에이전트 발전의 중요한 촉매제 역할을 하고 있습니다. 최근 오픈소스 LLM과 개발 도구의 증가는 더 많은 개발자와 연구자들이 AI 에이전트 기술에 접근할 수 있게 했습니다2.
Dflow와 같은 오픈소스 Python 툴킷은 과학자들이 간단한 프로그래밍 인터페이스로 복잡한 AI 워크플로우를 구성할 수 있게 해주며, 이는 특히 분산된 이기종 인프라에서 강력한 이점을 제공합니다6.
그러나 오픈소스의 양면성도 고려해야 합니다. 혁신적인 기술이 사회에 해로운 목적으로 오용될 수 있는 위험이 있기 때문입니다2. 따라서 책임 있는 AI 개발과 배포가 더욱 중요해지고 있습니다.
국제적 경쟁과 협력의 AI 생태계
AI 에이전트 기술 발전은 국가 간 경쟁과 협력의 역학 관계 속에서 진행되고 있습니다. 특히 미국과 중국의 AI 기술 경쟁이 두드러지고 있으며, 일본, 프랑스, 한국 등 여러 국가들도 독자적인 AI 생태계를 구축하기 위해 노력하고 있습니다.
중국의 엔지니어링 효율성
중국은 뛰어난 엔지니어와 연구자들을 보유하고 있으며, 이는 미국을 비롯한 다른 국가들이 주목해야 할 부분입니다. 특히 하드웨어 최적화와 대규모 시스템 통합 분야에서 중국의 접근 방식은 많은 시사점을 제공합니다.
오픈소스 기반 혁신의 중요성
다양한 국가에서 진행되고 있는 AI 에이전트 연구는 오픈소스 기술과 투명한 혁신을 중심으로 발전하고 있습니다. 특히 사회적 영향을 위한 오픈소스 AI 인프라 개발은 개발도상국의 연구자, 사회적 기업, 공공기관이 AI 솔루션을 개발하고 배포하는 데 필요한 도구와 전문 지식에 대한 접근성을 높이는 데 기여하고 있습니다4.
AI 에이전트의 미래: 다양한 분야에서의 혁신
AI 에이전트 기술은 앞으로 다양한 분야에서 혁신적인 응용 프로그램을 창출할 것으로 기대됩니다. 특히 다음 영역에서의 발전이 주목됩니다:
1. 로보틱스와 제조업
AI 에이전트는 로봇 공학과 제조업에서 자율 시스템의 핵심이 될 것입니다. 특히:
- 스마트 팩토리 환경에서의 자율 의사 결정
- 예측 유지보수를 통한 장비 효율성 증대
- 유연한 생산 라인 재구성 가능
- 인간-로봇 협업 최적화
이러한 발전은 제조업의 생산성과 안전성을 크게 향상시킬 것입니다.
2. 우주 탐사
우주 탐사에서 AI 에이전트는 결정적인 역할을 할 것입니다:
- 지구와의 통신 지연이 있는 환경에서 자율적인 의사 결정
- 예상치 못한 상황에 대한 적응형 대응
- 자원 제약 환경에서의 최적화된 운영
- 탐사 데이터의 실시간 분석 및 우선순위 지정
이러한 기능은 먼 거리와 극한 환경에서의 우주 탐사를 더욱 효과적으로 만들 것입니다.
3. 국방 및 안보
국방 분야에서 AI 에이전트는 전략적인 역할을 담당할 것입니다:
- 복잡한 시나리오에서의 상황 인식 개선
- 위협 감지 및 대응의 자동화
- 물류 및 공급망 최적화
- 사이버 보안 강화
이러한 적용은 국가 안보를 강화하는 동시에 인간 운영자의 부담을 줄일 수 있습니다.
결론: AI 에이전트가 가져올 미래
AI 에이전트 기술은 현재 중요한 발전 단계에 있습니다. 코딩, 고객 지원, 영업과 같은 영역에서 이미 가시적인 성과를 보이고 있지만, 여전히 복잡한 시스템 통합과 오류 누적 문제와 같은 과제가 남아 있습니다.
오픈소스 AI 클라우드와 같은 새로운 인프라 스택의 등장과 다양한 국가에서의 하드웨어 및 엔지니어링 효율성 향상 노력은 AI 에이전트 기술의 발전 가능성을 더욱 높이고 있습니다. 특히 로보틱스, 우주 탐사, 국방, 제조업 등 다양한 분야에서 혁신적인 응용 프로그램이 등장할 것으로 기대됩니다.
궁극적으로 AI 에이전트 기술이 성공하기 위해서는 인간 중심의 설계와 책임 있는 개발이 필수적입니다. 기술의 발전과 함께 윤리적, 사회적 영향에 대한 고려가 함께 이루어질 때, AI 에이전트는 진정한 의미에서 인간 사회에 기여할 수 있을 것입니다.
여러분은 어떻게 생각하시나요? AI 에이전트가 가장 큰 영향을 미칠 분야는 어디일까요? 또는 AI 에이전트 도입 시 가장 중요하게 고려해야 할 요소는 무엇일까요? 댓글로 여러분의 생각을 공유해 주세요!
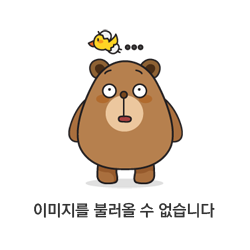
태그: #AI에이전트, #인공지능, #머신러닝, #클라우드컴퓨팅, #오픈소스AI, #로보틱스, #AI인프라, #자율시스템, #LLM, #기술혁신, #디지털전환, #스마트기술, #소프트웨어개발, #미래기술, #국제기술경쟁
🤖 2025 AI Agent Revolution: Exploring Challenges and Possibilities
As AI agent technology rapidly evolves, companies are marking 2025 as the 'Year of AI Agents.' From coding and customer support to applications in physical environments, we examine the current state and future possibilities of AI agents to understand the core of technological advancement.
The Current State of AI Agents: Achievements and Limitations
Today, AI agent technology is making remarkable progress. What exactly is an AI agent? From an engineering perspective, an AI agent is understood as an autonomous system or Large Language Model (LLM) that determines and performs actions on its own. Currently, businesses are moving beyond building AI infrastructure to focus on developing practical AI applications.
Application Cases in Business Environments
AI agent technology is particularly prominent in three key areas:
- Coding Support: Automated code generation and optimization to enhance developer productivity
- Customer Support: Building intelligent customer response systems available 24/7
- Sales Support: Improving sales processes through data-driven insights
Looking at real cases, companies like Maven AGI are achieving seamless integration of AI agents with existing systems, significantly saving time and costs while creating tangible business value. This represents not just technology adoption but innovation across entire business processes.
Practical Limitations of AI Agent Technology
Despite impressive advances, AI agent technology still faces significant limitations:
- Limited Verifiability: While text and image domains show high repeatability and verifiability, applications in physical environments remain limited.
- Workflow Integration Challenges: Integration is difficult in fields requiring access to informal data, such as robotics and warehouse management.
- Delayed ROI Realization: While data and workflow integration are progressing, it takes more time to achieve actual return on investment for businesses5.
According to Daniel Pelling's 'Physical AI' theory, applying AI agents in real environments requires establishing reward mechanisms. However, implementing such reward systems in the real world is much more complex than in digital environments.
The Complexity of Consumer Experience: Real-life Applications of AI Agents
When AI agents move beyond business environments into ordinary consumers' lives, the resulting issues become even more complex. Even seemingly simple tasks actually require consideration of numerous variables and options.
Flight Booking: A Good Example of Complexity
Consider the simple task of booking a flight. In this process, an AI agent must:
- Select appropriate airlines
- Identify optimal airports and stopovers
- Determine time slots that match personal preferences
- Balance price and convenience
- Decide on details like seat type, baggage options, mileage application, etc.
All these decisions must be handled perfectly for a satisfactory consumer experience. The problem is that AI agents are complex systems, and small errors accumulating over 50 steps can significantly degrade the user experience.
The Problem of Error Accumulation
One of the biggest challenges for AI agents is the error accumulation problem. A system with 95% accuracy at each step may see significantly reduced accuracy in the final result after going through 50 steps. This issue is particularly pronounced in environments requiring real-time decisions.
"The true value of AI agent systems lies not in the accuracy of individual tasks, but in how smoothly they complete the entire process."
Even in markets dominated by large companies, small businesses and startups have opportunities to present innovative solutions to these problems. A promising approach is to target niche markets through strategic collaboration with large companies.
The Evolution of AI Infrastructure: The Role of Cloud Platforms and Open Source
The future of AI agent technology depends on robust infrastructure. Cloud computing technology, in particular, is accelerating AI agent development by providing the infrastructure needed to train, deploy, and scale AI models5.
The Importance of Cloud-Based AI Development Environments
Major cloud providers such as Amazon Web Services (AWS), Microsoft Azure, and Google Cloud offer various services for generative AI, greatly enhancing the speed of AI agent development for businesses. These platforms provide:
- High-Performance Computing (HPC) resources
- Flexible scaling through serverless architecture
- Real-time processing via edge computing
- AI-specialized storage solutions
These features form the core foundation for AI agent development5.
The Duality of Open Source Ecosystems
Open source serves as an important catalyst for AI agent advancement. The recent increase in open-source LLMs and development tools has allowed more developers and researchers to access AI agent technology2.
Open-source Python toolkits like Dflow enable scientists to configure complex AI workflows with simple programming interfaces, providing particular advantages in distributed heterogeneous infrastructures6.
However, the duality of open source must also be considered. There is a risk that innovative technologies could be misused for harmful purposes2. Therefore, responsible AI development and deployment are becoming increasingly important.
International Competition and Cooperation in the AI Ecosystem
The advancement of AI agent technology is progressing within the dynamics of competition and cooperation between nations. The AI technology competition between the US and China is particularly prominent, while countries like Japan, France, and Korea are also working to build their own AI ecosystems.
China's Engineering Efficiency
China possesses excellent engineers and researchers, which is an aspect that other countries, including the US, should pay attention to. China's approach to hardware optimization and large-scale system integration provides many insights.
The Importance of Open Source-Based Innovation
AI agent research in various countries is developing around open-source technology and transparent innovation. In particular, the development of open-source AI infrastructure for social impact is helping to increase access to tools and expertise needed by researchers, social enterprises, and public institutions in developing countries to develop and deploy AI solutions4.
The Future of AI Agents: Innovation Across Various Fields
AI agent technology is expected to create innovative applications across various fields in the future. Developments in the following areas are particularly noteworthy:
1. Robotics and Manufacturing
AI agents will become the core of autonomous systems in robotics and manufacturing. In particular:
- Autonomous decision-making in smart factory environments
- Enhanced equipment efficiency through predictive maintenance
- Flexible production line reconfiguration
- Human-robot collaboration optimization
These advances will greatly improve productivity and safety in manufacturing.
2. Space Exploration
AI agents will play a decisive role in space exploration:
- Autonomous decision-making in environments with communication delays with Earth
- Adaptive responses to unexpected situations
- Optimized operations in resource-constrained environments
- Real-time analysis and prioritization of exploration data
These capabilities will make space exploration more effective in distant and extreme environments.
3. Defense and Security
In the defense sector, AI agents will play a strategic role:
- Improved situational awareness in complex scenarios
- Automation of threat detection and response
- Logistics and supply chain optimization
- Enhanced cybersecurity
These applications can strengthen national security while reducing the burden on human operators.
Conclusion: The Future Brought by AI Agents
AI agent technology is currently at a critical stage of development. While already showing visible results in areas such as coding, customer support, and sales, challenges like complex system integration and error accumulation problems remain.
The emergence of new infrastructure stacks such as open-source AI cloud and efforts to improve hardware and engineering efficiency in various countries are further increasing the development potential of AI agent technology. Innovative applications are expected to emerge particularly in robotics, space exploration, defense, and manufacturing.
Ultimately, human-centered design and responsible development are essential for AI agent technology to succeed. When technological advancement is accompanied by consideration of ethical and social impacts, AI agents can truly contribute to human society in a meaningful way.
What do you think? In which areas will AI agents have the greatest impact? Or what are the most important factors to consider when adopting AI agents? Please share your thoughts in the comments!
Tags: #AIAgent, #ArtificialIntelligence, #MachineLearning, #CloudComputing, #OpenSourceAI, #Robotics, #AIInfrastructure, #AutonomousSystems, #LLM, #TechnologicalInnovation, #DigitalTransformation, #SmartTechnology, #SoftwareDevelopment, #FutureTechnology, #InternationalTechCompetition
Citations:
- https://arxiv.org/html/2406.07736v3
- https://arxiv.org/html/2403.06537v2
- https://arxiv.org/abs/1912.10344
- https://openreview.net/forum?id=RKUtVmLDpo
- https://arxiv.org/html/2412.06044v1
- https://arxiv.org/abs/2404.18392
- https://www.semanticscholar.org/paper/af1523796f1068605fba7d66a037a0ad2a257a94
- https://www.semanticscholar.org/paper/aadbc14c8880aab937fa0047d2ba26893589c55d
- https://www.semanticscholar.org/paper/c02903dda56f330599ad1759f1331cef4d84b541
'Agentic AI' 카테고리의 다른 글
미래를 바꿀 AI 에이전트 기술, 어디까지 왔나? (Anthropic의 Claude와 Manus AI 협업 사례) (1) | 2025.03.22 |
---|---|
에이전트 오케스트레이션의 새 시대: Adobe의 AI 혁신이 가져올 미래 (0) | 2025.03.20 |
🚀 AI 에이전트의 미래로 도약하기: 2025년을 준비하는 효과적인 구축 전략 (0) | 2025.03.20 |
2025년 AI 혁명의 물결에서 부를 창출하는 5가지 전략: 당신의 기회는 지금입니다 (0) | 2025.03.18 |
🤖 코딩 한 줄 없이 나만의 AI 비서 만들기: n8n으로 구현하는 똑똑한 자동화 에이전트 (0) | 2025.03.17 |