배포된 AI 모델이 처음의 완벽한 성능을 유지하지 못하고 시간이 지남에 따라 예상치 못한 결과를 내놓는 경우가 너무나 많습니다. 특히 개발 환경에서는 문제없이 작동하던 모델이 실제 환경에 적용되면 정확도가 떨어지거나 부적절한 내용을 생성하는 경우, 이는 사용자 신뢰도 하락과 심각한 비즈니스 손실로 이어질 수 있습니다. AI 모델의 지속적인 신뢰성 확보는 모든 AI 시스템의 성공을 위한 핵심 요소입니다.
모델 드리프트의 이해: 왜 AI 모델은 시간이 지나면 성능이 저하될까?
모델 드리프트는 데이터 또는 입력 변수와 출력 변수 간의 관계 변화로 인해 머신 러닝 모델의 성능이 저하되는 현상을 말합니다. 이는 잘못된 의사 결정과 예측을 초래할 수 있어 AI 시스템의 신뢰성에 직접적인 위협이 됩니다1.
드리프트의 유형은 크게 세 가지로 나눌 수 있습니다:
개념 드리프트(Concept Drift)
개념 드리프트는 입력 변수와 대상 변수 사이의 관계가 변화할 때 발생합니다. 예를 들어, COVID-19 팬데믹으로 인해 소비자 행동이 급격히 변화하면서 기존의 구매 패턴 예측 모델이 무용지물이 된 경우가 있었습니다1.
이러한 개념 드리프트는 다음과 같은 방식으로 발생할 수 있습니다:
- 계절성 변화: 날씨 변화에 따른 제품 구매 패턴 변화
- 갑작스러운 사회적 변화: 팬데믹이나 새로운 기술 등장으로 인한 행동 변화
- 점진적 변화: 스팸과 해킹 기술의 진화처럼 천천히 진행되는 변화
데이터 드리프트(Data Drift)
데이터 드리프트(공변량 변화라고도 함)는 입력 데이터의 기본 분포가 변경될 때 발생합니다. 예를 들어, 원래 젊은 층을 대상으로 한 웹사이트가 노년층에게 인기를 얻게 되면, 사용자 행동 예측 모델이 제대로 작동하지 않을 수 있습니다1.
업스트림 데이터 변경
이는 데이터 파이프라인 자체가 변경될 때 발생합니다. 예를 들어, 측정 단위 변경(USD에서 유로로, 킬로미터에서 마일로)은 모델이 이러한 변경을 인식하도록 설계되지 않았다면 성능에 심각한 영향을 줄 수 있습니다1.
AI 모델 신뢰성 확보를 위한 3가지 핵심 전략
AI 모델이 배포 후에도 안정적으로 작동하고 예상치 못한 결과를 최소화하기 위해 다음 세 가지 전략을 적용할 수 있습니다.
1. 실제 데이터(Ground Truth)와 모델 출력 비교
모델이 현실 세계에서 제대로 작동하는지 확인하는 가장 기본적인 방법은 실제 데이터(ground truth)와 모델 출력을 비교하는 것입니다. Ground truth는 AI 모델의 학습과 성능 평가의 기준이 되는 이상적인 정답을 의미합니다.
실제 활용 사례:
- 예측 모델: 고객 이탈을 예측했는데, 실제로 이탈하지 않은 고객을 이탈할 것으로 잘못 예측했다면, 모델의 정확도에 문제가 있다는 신호입니다.
- 생성 모델: 이메일 작성 AI가 특정 프롬프트에 대해 사람이 작성한 이메일과 전혀 다른 내용을 생성한다면 모델을 재조정해야 합니다.
실무 적용 팁:
- 정기적 데이터 수집: 실제 환경에서 데이터를 수집하고 모델의 출력과 비교하는 시스템을 구축하세요.
- 성능 지표 정의: 정확도, 정밀도, 재현율 등 모델 성능을 측정할 수 있는 명확한 지표를 설정하세요.
- 재훈련 임계값 설정: 성능이 특정 임계값 이하로 떨어지면 자동으로 재훈련을 트리거하는 시스템을 구현하세요.
"지속적으로 수신되는 새로운 데이터 포인트(새로운 변형, 새로운 패턴, 새로운 트렌드 등)는 과거 데이터만으로는 포착할 수 없습니다. AI 모델의 훈련이 수신 데이터와 일치하지 않으면 해당 데이터를 정확하게 해석할 수 없습니다."1
2. 개발 환경과 배포 환경에서의 출력 비교
모델이 개발 단계와 배포 단계에서 다르게 작동하는 현상을 감지하려면 두 환경 간의 출력을 정기적으로 비교해야 합니다. 이를 통해 환경 차이에서 발생하는 문제를 빠르게 발견할 수 있습니다.
실제 활용 사례:
- 수치적 출력: 개발 단계에서 평균 4%의 이탈률을 예측했는데, 실제 배포 후 평균 이탈률이 0.4%로 나타난다면 환경 간 차이가 존재하는 것입니다.
- 텍스트 출력: 10학년 학생 수준의 대화를 하도록 설계된 챗봇이 배포 후 갑자기 이해하기 어려운 표현을 사용한다면 즉시 조치가 필요합니다.
실무 적용 팁:
- 샤도우 배포(Shadow Deployment): 실제 환경에 모델을 배포하기 전에 실제 트래픽을 복제하여 테스트 환경에서 모델 성능을 확인하세요.
- A/B 테스트: 새 모델을 일부 사용자에게만 적용하여 기존 모델과 성능을 비교하세요12.
- 자동화된 모니터링 도구 활용: Prometheus, Grafana, Evidently AI와 같은 모니터링 도구를 사용하여 모델 성능을 실시간으로 추적하세요7.
"드리프트를 신속하게 감지하고 완화하지 않으면 드리프트가 더 심해져 운영에 대한 피해가 커질 수 있습니다. 드리프트 감지 기능을 통해 조직은 모델로부터 정확한 아웃풋을 지속적으로 받을 수 있습니다."1
3. 출력에 플래그(flag) 또는 필터(filter) 적용
AI 모델은 때로 의도하지 않은 민감 정보나 부적절한 콘텐츠를 생성할 수 있습니다. 이런 위험을 방지하려면 특정 조건에 따라 출력을 자동으로 필터링하거나 플래그를 지정하는 시스템을 구축해야 합니다.
실제 활용 사례:
- 개인정보 보호: 사회보장번호, 전화번호 등 민감 정보가 포함된 출력은 자동으로 플래그 처리하여 외부로 유출되지 않도록 합니다.
- 유해 콘텐츠 차단: 증오 발언, 욕설, 학대 등이 포함된 콘텐츠는 필터링하여 사용자에게 전달되지 않도록 합니다.
실무 적용 팁:
- 콘텐츠 정책 수립: 어떤 종류의 콘텐츠가 허용되고 어떤 것이 차단되어야 하는지 명확한 정책을 수립하세요.
- 입출력 분류기 구현: 입력 분류기와 출력 분류기를 모두 구현하여 두 단계에서 필터링을 수행하세요4.
- 인간 검토 프로세스: 플래그가 지정된 콘텐츠는 사람이 검토하여 추가 조치를 취하도록 프로세스를 설계하세요.
"출력 분류기는 모델 출력을 필터링하여 안전 정책을 위반하는 생성된 콘텐츠를 포착합니다. 콘텐츠 거부 행동을 주의 깊게 모니터링하면 입력을 보강하거나 개선하는 데 사용할 수 있는 새로운 유형의 필터를 적용할 수 있습니다."4
결론: AI 모델 관리는 지속적인 여정
AI 모델은 한 번 개발하고 배포한다고 끝나는 것이 아닙니다. 세상은 끊임없이 변화하기 때문에 그 세상을 이해하는 데 사용되는 모델도 지속적으로 검토하고 업데이트해야 합니다1. 위에서 소개한 세 가지 전략—실제 데이터와의 비교, 개발 및 배포 환경 간 출력 비교, 출력 필터링—을 적용하면 AI 모델의 신뢰성과 안전성을 크게 향상시킬 수 있습니다.
다음 질문들을 통해 여러분의 AI 시스템에 이러한 전략을 어떻게 적용할 수 있을지 고민해 보세요:
- 현재 운영 중인 AI 모델의 성능을 얼마나 자주 모니터링하고 있나요?
- 모델 드리프트를 감지하기 위한 자동화된 시스템이 구축되어 있나요?
- 민감 정보와 유해 콘텐츠를 필터링하기 위한 메커니즘은 얼마나 효과적인가요?
- 모델 재훈련의 주기와 기준이 명확하게 정의되어 있나요?
AI 모델의 지속적인 관리와 모니터링은 단순한 비용이 아닌 필수적인 투자입니다. 이러한 투자는 모델의 성능을 유지하고, 사용자 신뢰를 구축하며, 궁극적으로 AI 시스템의 장기적 성공을 보장하는 데 핵심적인 역할을 합니다.
#AI모델 #모델드리프트 #신뢰성 #AI안전성 #데이터과학 #머신러닝 #모델관리 #출력필터링 #AI윤리 #배포환경 #예측정확도 #개인정보보호 #유해콘텐츠차단 #그라운드트루스 #모델모니터링 #개념드리프트 #데이터드리프트 #앙상블학습 #재훈련전략 #모델성능
Building Trustworthy AI: 3 Key Strategies to Prevent Model Drift and Unsafe Outputs
AI models that perform perfectly in development environments often produce unexpected results after deployment. When accuracy drops or inappropriate content is generated in real-world environments, this can lead to decreased user trust and serious business losses. Maintaining continuous AI model reliability is a critical factor in the success of any AI system.
Understanding Model Drift: Why AI Models Degrade Over Time
Model drift refers to the degradation of machine learning model performance due to changes in data or relationships between input and output variables. This can lead to incorrect decisions and predictions, posing a direct threat to the reliability of AI systems1.
Drift can be categorized into three main types:
Concept Drift
Concept drift occurs when the relationship between input variables and target variables changes. For example, during the COVID-19 pandemic, consumer behavior changed dramatically, rendering existing purchase pattern prediction models useless1.
Concept drift can occur in several ways:
- Seasonal changes: Changes in product purchasing patterns due to weather fluctuations
- Sudden social changes: Behavioral changes caused by pandemics or emerging technologies
- Gradual changes: Slowly evolving changes, like the evolution of spam and hacking techniques
Data Drift
Data drift (also called covariate shift) occurs when the underlying distribution of input data changes. For instance, if a website originally targeting young people becomes popular among older adults, user behavior prediction models may not function properly1.
Upstream Data Changes
This occurs when the data pipeline itself changes. For example, changes in measurement units (from USD to euros, kilometers to miles) can severely impact model performance if the model isn't designed to recognize these changes1.
3 Key Strategies for Ensuring AI Model Reliability
To ensure AI models operate stably after deployment and minimize unexpected outcomes, you can apply these three strategies.
1. Compare Model Outputs with Ground Truth Data
The most fundamental way to check if your model is working properly in the real world is to compare its outputs with ground truth data. Ground truth refers to the ideal answers that serve as the standard for training and evaluating the performance of AI models.
Real-world Applications:
- Predictive Models: If your model incorrectly predicts that a customer will churn when they actually don't, it signals an accuracy problem.
- Generative Models: If an email-writing AI generates content completely different from what a human would write for a specific prompt, the model needs adjustment.
Practical Implementation Tips:
- Regular Data Collection: Build a system to collect real-world data and compare it with your model's outputs.
- Performance Metric Definition: Establish clear metrics such as accuracy, precision, and recall to measure model performance.
- Retraining Threshold Setting: Implement a system that automatically triggers retraining when performance falls below a specific threshold.
"Continuously incoming new data points (new variations, patterns, trends, etc.) cannot be captured by historical data alone. If AI model training doesn't match the incoming data, the model cannot accurately interpret that data."1
2. Compare Outputs Between Development and Deployment Environments
To detect instances where models behave differently in development versus deployment stages, regular comparison of outputs between these environments is essential. This helps quickly identify problems arising from environmental differences.
Real-world Applications:
- Numerical Outputs: If you predicted an average churn rate of 4% in development but see 0.4% after deployment, there's a difference between environments.
- Text Outputs: If a chatbot designed to communicate at a 10th-grade level suddenly starts using difficult expressions after deployment, immediate action is needed.
Practical Implementation Tips:
- Shadow Deployment: Before deploying your model to the production environment, test its performance in a test environment using replicated real traffic.
- A/B Testing: Apply new models to only a subset of users to compare performance with existing models12.
- Automated Monitoring Tools: Use monitoring tools like Prometheus, Grafana, and Evidently AI to track model performance in real-time7.
"If drift is not quickly detected and mitigated, it can worsen and increase operational damage. Drift detection capabilities allow organizations to continuously receive accurate outputs from their models."1
3. Apply Flags or Filters to Outputs
AI models sometimes generate unintended sensitive information or inappropriate content. To prevent these risks, you should build systems that automatically filter outputs or flag them based on specific conditions.
Real-world Applications:
- Privacy Protection: Automatically flag outputs containing sensitive information like social security numbers and phone numbers to prevent external leakage.
- Harmful Content Blocking: Filter content containing hate speech, profanity, or abuse to prevent it from reaching users.
Practical Implementation Tips:
- Content Policy Establishment: Establish clear policies on what types of content are allowed and what should be blocked.
- Input and Output Classifier Implementation: Implement both input and output classifiers to perform filtering at two stages4.
- Human Review Process: Design a process where flagged content is reviewed by humans for additional action.
"Output classifiers filter model outputs to capture generated content that violates safety policies. Carefully monitoring content rejection behavior allows for the application of new types of filters that can be used to augment or improve inputs."4
Conclusion: AI Model Management is a Continuous Journey
AI models don't end with development and deployment. Because the world is constantly changing, models used to understand that world must be continuously reviewed and updated1. By applying the three strategies introduced above—comparing with real data, comparing outputs between development and deployment environments, and filtering outputs—you can greatly improve the reliability and safety of AI models.
Consider how you can apply these strategies to your AI systems by asking the following questions:
- How often do you monitor the performance of your currently operating AI models?
- Do you have automated systems in place to detect model drift?
- How effective are your mechanisms for filtering sensitive information and harmful content?
- Are your model retraining cycles and criteria clearly defined?
Continuous management and monitoring of AI models is not merely a cost but an essential investment. This investment plays a crucial role in maintaining model performance, building user trust, and ultimately ensuring the long-term success of AI systems.
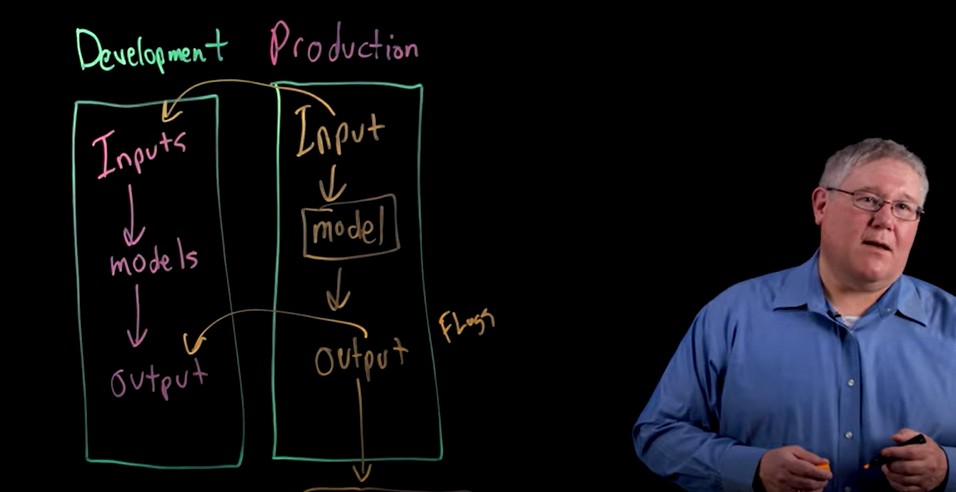
'DeepResearch' 카테고리의 다른 글
인간 수준의 AI를 향한 여정: 얀 르쿤이 AI Action Summit 2025에서 제시한 비전 (0) | 2025.03.14 |
---|---|
Ground Truth 데이터: AI와 머신 러닝 모델의 성공을 좌우하는 핵심 요소 (0) | 2025.03.14 |
AI와 창의성의 공존: 대체가 아닌 협력의 새로운 시대 (0) | 2025.03.13 |
단순 대화창에서 지능형 비서로: 챗봇과 AI 어시스턴트의 핵심 차이점 (0) | 2025.03.13 |
인공지능의 내면: AI는 정말 자의식을 가질 수 있을까? (0) | 2025.03.12 |