에이전트와 워크플로우의 명확한 구분을 통해 AI 개발의 효율성을 극대화하는 방법을 알아봅니다.
Agentic AI는 최근 인공지능 분야에서 가장 뜨거운 화두 중 하나입니다. 하지만 '에이전트'라는 용어가 무분별하게 사용되면서 개념적 혼란이 발생하고 있습니다. 이 글에서는 Agentic AI의 핵심 개념인 '에이전트'와 '에이전트 워크플로우'의 차이점을 명확히 설명하고, 실제 개발 환경에서 이를 효과적으로 활용하는 방법에 대해 알아보겠습니다. 비결정론적 접근과 결정론적 접근의 차이를 이해하면, 문제 상황에 맞는 최적의 AI 솔루션을 구현할 수 있습니다.
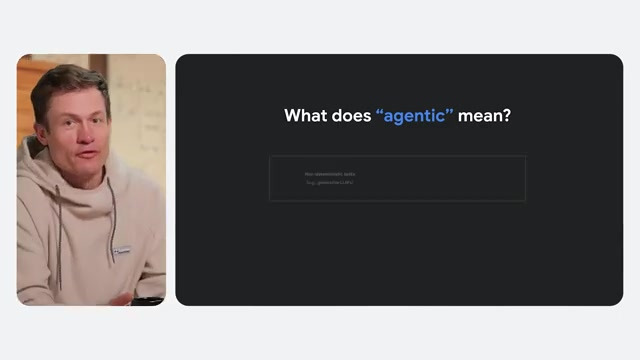
Agentic AI의 개념과 중요성
에이전트에 대한 명확한 정의의 필요성
Agentic AI 시즌 2에서는 개발자들에게 친숙한 용어를 사용하여 다양한 AI 관련 개념을 다룹니다. 이전 시즌에서 생성 AI의 기반이 되는 요소들을 살펴봤다면, 이번 시즌은 '에이전틱'에 초점을 맞춥니다. 특히 '에이전트'라는 용어에 대한 명확한 정의가 필요하다는 인식 하에, 이번 시즌을 통해 이 개념을 심도 있게 다룰 예정입니다1.
최근 에이전틱 행동에 관한 다양한 연구와 출판물이 증가하고 있습니다. 이러한 흐름은 AI 개발 및 응용 분야에서 에이전트 개념의 중요성이 점점 더 부각되고 있음을 보여줍니다5.
에이전틱 AI의 정의
에이전틱 AI는 비결정론적인 생성 AI 언어 모델을 활용하여 예측이 어려운 결과를 생성하는 시스템을 의미합니다. 이러한 시스템은 작업과 워크플로우를 결합하여 특정 목표를 향해 나아가는 AI 에이전트를 생성할 수 있습니다2.
최근 연구에 따르면, 대규모 언어 모델(LLM)을 기반으로 한 다중 에이전트 프레임워크는 자동화된 계획 및 작업 실행에서 뛰어난 성과를 보여주고 있습니다3. 이러한 에이전트 시스템은 단순한 정보 처리를 넘어 복잡한 추론과 의사결정까지 수행할 수 있는 역량을 갖추고 있습니다.
에이전트와 워크플로우의 핵심 차이점
결정론적 워크플로우 vs. 비결정론적 에이전트
워크플로우의 특징:
- 결정론적이고 예측 가능한 방식으로 작업 처리
- 의사결정과 결과에 더 집중하여 높은 예측 가능성 제공
- 명확하게 정의된 단계와 프로세스를 따름
에이전트의 특징:
- 비결정론적 방식으로 작업 수행
- 목표 달성을 위한 자율적 의사결정 능력 보유
- 다양한 상황에 적응하고 유연하게 대응3
실제 연구에서도 워크플로우는 활동-정점(AOV) 그래프로 정의되며, LLM 에이전트가 역사적 성과와 이전 AOV를 기반으로 동적으로 하위 작업 할당을 조정함으로써 지속적인 워크플로우 개선이 가능합니다3. 이는 워크플로우가 체계적이고 구조화된 접근 방식을 취한다는 것을 보여줍니다.
실제 적용 사례를 통한 이해
워크플로우 적용 사례: 청구서 처리
청구서 처리는 워크플로우 적용의 좋은 예입니다. 이 과정에서 LLM을 활용해 청구서의 데이터를 분석하고 양식에 입력하는 방식을 취합니다. 사용자는 정보를 검토한 후 양식을 처리 도구에 업로드하는 역할을 담당합니다1. 이 접근법은 명확한 단계와 예측 가능한 결과를 제공합니다.
에이전트 적용 사례: 소프트웨어 개발
AI 에이전트는 목표만 제시하면 자율적으로 작업을 수행합니다. 예를 들어, "게임 웹사이트를 만들어줘"라는 요청에 에이전트는 설계, 코딩, 테스트 등 일련의 과정을 스스로 판단하고 수행합니다5. 최근 연구인 AFlow는 몬테카를로 트리 검색을 활용하여 효율적으로 워크플로우 공간을 탐색하고, 코드 수정, 트리 구조 경험, 실행 피드백을 통해 워크플로우를 반복적으로 개선합니다5.
에이전트적 AI의 핵심 작동 원리
자율적 프로세스의 3단계
에이전트는 다음과 같은 자율적 프로세스를 통해 작업을 수행합니다:
- 작업 정의: 목표와 수행해야 할 작업을 명확히 정의
- 작업 수행: 자율적으로 작업을 실행하고 필요에 따라 결정 내림
- 반성 과정: 결과가 초기 목표에 부합하는지 평가하고 필요시 조정6
이러한 접근 방식은 의사결정의 주체성을 강화하며, 인간-AI 상호작용에 대한 이해를 증진시킵니다4. 대화형 인간-AI 상호작용(CHAI) 연구에서도 에이전틱 워크플로우를 통해 맥락화, 목표 공식화 등의 단계를 통해 대화를 안내하는 방법이 제안되고 있습니다4.
실제 비즈니스 환경에서의 분석
비즈니스 환경에서는 다양한 요소를 세부적으로 분석하고 구분하는 것이 중요합니다. 의료 분야에서는 LLaMA 3 8B를 사용한 완전 자동화된 다중 에이전트 AI 워크플로우가 임상 메모에서 인지적 문제를 식별하는 데 높은 성능(F1-점수 0.90)을 달성했습니다6. 이는 에이전틱 AI가 실제 데이터에서 중요한 통찰력을 추출하는 데 효과적임을 보여줍니다.
에이전트와 워크플로우의 선택 기준
모든 문제에 에이전트가 필요한 것은 아니다
AI 에이전트를 생성하거나 에이전트적 워크플로우를 사용할 때, 모든 문제에 AI 에이전트가 필요한 것은 아닙니다. 대부분의 경우, 에이전트적 워크플로우가 더 적합할 수 있습니다1.
워크플로우가 적합한 경우:
- 예측 가능하고 구조화된 프로세스가 필요할 때
- 오류 발생 시 심각한 결과를 초래할 수 있는 중요한 작업
- 명확한 규칙과 절차를 따라야 하는 규제가 많은 산업의 업무2
에이전트가 적합한 경우:
- 복잡하고 다양한 의사결정이 필요한 상황
- 새로운 상황에 적응해야 하는 유연한 접근 방식이 필요할 때
- 목표가 명확하지만 달성 방법에 여러 가지 가능성이 있을 때5
최적의 솔루션 선택하기
단일 LLM 단계 또는 소수의 LLM 단계를 기존의 결정론적 워크플로우에 추가하는 것만으로도 상당한 이점을 얻을 수 있습니다1. 이러한 하이브리드 접근 방식은 예측 가능성과 유연성의 균형을 제공합니다.
에이전틱 에이전트를 구축할 경우, 그것이 인간의 선택과 다를 수 있다는 위험을 항상 고려해야 합니다. 중요한 의사결정이 필요한 상황에서는 의도적으로 인간을 프로세스에 포함시키고, 보다 결정론적으로 행동하는 방향으로 작업을 축소하는 것이 바람직할 수 있습니다2.
Agentic AI의 실용적 구현과 미래 전망
모듈화된 접근 방식의 중요성
최근 연구에서는 워크플로우 설계에 모듈성을 강조하는 경향이 있습니다. 병렬성과 의존성 복잡성을 평가하여 효율적인 워크플로우를 구축하는 것이 중요합니다3. 이러한 모듈화된 접근 방식은 하위 작업의 효율적인 동시 실행, 효과적인 목표 달성, 오류 허용 범위 향상을 제공합니다.
자동화된 워크플로우 생성의 발전
AFlow와 같은 자동화된 프레임워크는 몬테카를로 트리 검색을 사용하여 코드로 표현된 워크플로우 공간을 효율적으로 탐색합니다. 이를 통해 코드 수정, 트리 구조 경험, 실행 피드백을 통해 워크플로우를 반복적으로 개선할 수 있습니다5. 이러한 접근 방식은 GPT-4o보다 4.55%의 비용으로 특정 작업에서 더 나은 성능을 달성할 수 있게 했습니다.
표준 운영 절차(SOP) 자동화
Agent-S는 LLM 기반 에이전트 워크플로우를 사용하여 표준 운영 절차(SOP)를 자동화하는 방법을 제안합니다. 이 아키텍처는 세 가지 작업별 LLM, 글로벌 액션 저장소(GAR), 실행 메모리 및 여러 환경으로 구성됩니다1. SOP 워크플로우는 단순한 논리적 텍스트 블록으로 작성됩니다. 현재 실행 메모리와 SOP를 기반으로 에이전트는 실행할 작업을 선택하고, 적절한 환경(사용자/API)과 상호 작용하여 관찰 및 피드백을 수집합니다.
결론: Agentic AI의 균형 잡힌 접근법
Agentic AI 분야는 빠르게 발전하고 있으며, 에이전트와 워크플로우의 차이점을 이해하는 것은 효과적인 AI 솔루션을 개발하는 데 필수적입니다. 모든 문제에 AI 에이전트가 필요한 것은 아니며, 많은 경우 기존 워크플로우에 LLM 기능을 통합하는 것만으로도 충분한 이점을 얻을 수 있습니다.
특히 중요한 것은 목표와 결과를 명확히 정의할 수 있는 경우, 에이전트의 자율성이 도움이 되는 작업에 에이전트를 활용하는 것입니다. 반면, 예측 가능성과 신뢰성이 중요한 작업에는 보다 구조화된 워크플로우 접근 방식을 고려해야 합니다.
앞으로 Agentic AI 분야는 더욱 발전하여 다양한 산업과 응용 분야에서 혁신을 이끌 것입니다. 개발자와 연구자들은 이러한 개념을 명확히 이해하고 적절히 적용함으로써 더 효과적이고 효율적인 AI 시스템을 구축할 수 있을 것입니다.
여러분은 어떤 프로젝트에 에이전트를 적용하고, 어떤 프로젝트에 워크플로우를 적용하는 것이 적합할까요? 자신의 프로젝트를 분석하고 최적의 접근 방식을 선택해보세요!
참고 자료 및 추가 리소스
- GitHub 링크를 통해 에이전트 및 에이전트 워크플로우 예시 코드를 확인하세요
- Jason과 함께하는 프롬프트 작업 시리즈를 통해 더 많은 내용을 학습할 수 있습니다
#AgenticAI #AIAgent #WorkflowAutomation #LLM #GenerativeAI #AIWorkflow #AISystemDesign #MachineLearning #AIDecisionMaking #AgentFramework #AIImplementation #SoftwareDevelopment #BusinessAutomation #TechInnovation #AIBestPractices
Understanding the Core of Agentic AI: Differences Between Agents and Workflows and Practical Application Strategies
Learn how to maximize AI development efficiency through a clear distinction between agents and workflows.
Agentic AI is one of the hottest topics in the field of artificial intelligence recently. However, conceptual confusion has arisen as the term 'agent' is being used indiscriminately. In this article, we will clearly explain the differences between 'agents' and 'agent workflows', the core concepts of Agentic AI, and explore how to effectively utilize them in actual development environments. By understanding the difference between non-deterministic and deterministic approaches, you can implement the optimal AI solution for problem situations.
Concept and Importance of Agentic AI
The Need for a Clear Definition of Agents
Agentic AI Season 2 deals with various AI-related concepts using developer-friendly terminology. While the previous season examined the elements that form the basis of generative AI, this season focuses on 'agentic'. In particular, with the recognition that a clear definition of the term 'agent' is needed, this season will delve deeply into this concept1.
Recently, there has been an increase in research and publications on agentic behavior. This trend shows that the importance of the agent concept is becoming increasingly prominent in the field of AI development and applications5.
Definition of Agentic AI
Agentic AI refers to systems that use non-deterministic generative AI language models to produce results that are difficult to predict. These systems can create AI agents that combine tasks and workflows to move toward specific goals2.
According to recent research, multi-agent frameworks based on Large Language Models (LLMs) are showing excellent performance in automated planning and task execution3. These agent systems have the capability to perform complex reasoning and decision-making beyond simple information processing.
Key Differences Between Agents and Workflows
Deterministic Workflows vs. Non-deterministic Agents
Characteristics of Workflows:
- Process tasks in a deterministic and predictable manner
- Focus more on decision-making and results, providing high predictability
- Follow clearly defined steps and processes
Characteristics of Agents:
- Perform tasks in a non-deterministic manner
- Possess autonomous decision-making ability to achieve goals
- Adapt to various situations and respond flexibly3
In actual research, workflows are defined as Activity-on-Vertex (AOV) graphs, and LLM agents can continuously improve workflows by dynamically adjusting subtask allocation based on historical performance and previous AOVs3. This demonstrates that workflows take a systematic and structured approach.
Understanding Through Real Application Cases
Workflow Application Case: Invoice Processing
Invoice processing is a good example of workflow application. In this process, LLMs are used to analyze invoice data and input it into forms. Users are responsible for reviewing the information and uploading the form to the processing tool1. This approach provides clear steps and predictable results.
Agent Application Case: Software Development
AI agents perform tasks autonomously when only the goal is presented. For example, when requested to "create a game website," the agent judges and performs a series of processes such as design, coding, and testing on its own5. Recent research like AFlow uses Monte Carlo Tree Search to efficiently explore the workflow space and iteratively improve workflows through code modification, tree structure experience, and execution feedback5.
Core Operating Principles of Agentic AI
Three Stages of Autonomous Process
Agents perform tasks through the following autonomous process:
- Task Definition: Clearly define goals and tasks to be performed
- Task Execution: Execute tasks autonomously and make decisions as needed
- Reflection Process: Evaluate whether the results align with the initial goals and make adjustments if necessary6
This approach strengthens the subjectivity of decision-making and enhances understanding of human-AI interaction4. In research on Conversational Human-AI Interaction (CHAI), methods have been proposed to guide conversations through phases such as contextualization and goal formulation via agentic workflows4.
Analysis in Real Business Environments
In business environments, it is important to analyze and distinguish various elements in detail. In the medical field, a fully automated multi-agent AI workflow using LLaMA 3 8B achieved high performance (F1-score of 0.90) in identifying cognitive issues in clinical notes6. This demonstrates that agentic AI is effective in extracting important insights from real data.
Selection Criteria for Agents and Workflows
Not All Problems Require Agents
When creating AI agents or using agentic workflows, not all problems require AI agents. In most cases, agentic workflows may be more suitable1.
When Workflows are Appropriate:
- When predictable and structured processes are needed
- Critical tasks where errors can lead to serious consequences
- Tasks in heavily regulated industries that must follow clear rules and procedures2
When Agents are Appropriate:
- Situations requiring complex and diverse decision-making
- When a flexible approach is needed to adapt to new situations
- When goals are clear but there are various possibilities for achievement5
Choosing the Optimal Solution
Significant benefits can be obtained by simply adding a single LLM step or a few LLM steps to existing deterministic workflows1. This hybrid approach provides a balance of predictability and flexibility.
When building agentic agents, the risk that they may differ from human choices should always be considered. In situations requiring important decisions, it may be desirable to intentionally include humans in the process and scale down tasks to behave more deterministically2.
Practical Implementation and Future Outlook of Agentic AI
Importance of Modularized Approach
Recent research tends to emphasize modularity in workflow design. It is important to build efficient workflows by evaluating parallelism and dependency complexity3. This modularized approach provides efficient concurrent execution of subtasks, effective goal achievement, and improved error tolerance.
Advancement of Automated Workflow Generation
Automated frameworks like AFlow efficiently explore code-represented workflow spaces using Monte Carlo Tree Search. This allows for iterative improvement of workflows through code modification, tree structure experience, and execution feedback5. This approach has enabled better performance on specific tasks at 4.55% of the cost compared to GPT-4o.
Standard Operating Procedure (SOP) Automation
Agent-S proposes a method to automate Standard Operating Procedures (SOPs) using LLM-based agent workflows. This architecture consists of three task-specific LLMs, a Global Action Repository (GAR), execution memory, and multiple environments1. SOP workflows are written as simple logical blocks of text. Based on the current execution memory and SOP, the agent selects actions to execute, interacts with appropriate environments (user/API) to collect observations and feedback.
Conclusion: Balanced Approach to Agentic AI
The field of Agentic AI is rapidly evolving, and understanding the differences between agents and workflows is essential for developing effective AI solutions. Not all problems require AI agents, and in many cases, integrating LLM capabilities into existing workflows can provide sufficient benefits.
Particularly important is utilizing agents for tasks where agent autonomy is helpful when goals and results can be clearly defined. Conversely, more structured workflow approaches should be considered for tasks where predictability and reliability are important.
In the future, the field of Agentic AI will continue to develop, leading innovation in various industries and application fields. Developers and researchers can build more effective and efficient AI systems by clearly understanding and appropriately applying these concepts.
Which projects would be suitable for applying agents, and which projects would be appropriate for applying workflows? Analyze your project and choose the optimal approach!
References and Additional Resources
- Check out agent and agent workflow example code through the GitHub link
- Learn more through the prompt work series with Jason
#AgenticAI #AIAgent #WorkflowAutomation #LLM #GenerativeAI #AIWorkflow #AISystemDesign #MachineLearning #AIDecisionMaking #AgentFramework #AIImplementation #SoftwareDevelopment #BusinessAutomation #TechInnovation #AIBestPractices
Citations:
- https://arxiv.org/abs/2503.15520
- https://arxiv.org/html/2501.07834v2/
- https://arxiv.org/abs/2501.07834
- https://arxiv.org/html/2501.18002v1
- https://arxiv.org/abs/2410.10762
- https://arxiv.org/abs/2502.01789
- https://www.semanticscholar.org/paper/772a055393a6aa758662317dbaa3d9a344c9ee79
- https://www.semanticscholar.org/paper/e35c4f09bf1cdca5bdeb5789a68b21ac99612d02
- https://www.semanticscholar.org/paper/3ee6b7849406c9e63a0a1f6222a444b46e1035bd
- https://www.semanticscholar.org/paper/58e5a52bdbeeb7b9cafaf0e9ae67a671211773f7
'Agentic AI' 카테고리의 다른 글
AI 에이전트 시대의 필수 프로토콜: MCP(모델 컨텍스트 프로토콜) 완벽 가이드 (0) | 2025.03.25 |
---|---|
【무료 설치 가이드】 Docker로 n8n과 MCP 설정하고 AI 자동화의 힘을 경험하세요! (0) | 2025.03.24 |
에이전트 AI 혁명: 여러 LLM을 조합한 스마트 솔루션 개발의 비밀 (0) | 2025.03.23 |
AI 에이전트와 사용자 경험(UX): 성공적인 상호작용을 위한 디자인의 중요성 (0) | 2025.03.23 |
PydanticAI로 구현하는 차세대 에이전틱 AI 시스템: 구조화된 데이터 처리의 새로운 지평 (0) | 2025.03.22 |