최근 AI 기술의 발전 속도가 기하급수적으로 증가하면서, 개발자와 기업들에게 흥미진진한 새로운 기회가 펼쳐지고 있습니다. NVIDIA GTC에서 발표된 혁신적인 로봇 기술부터 Baidu의 전략적 AI 모델 접근법, Chain-of-Thought 추론의 신뢰성 문제까지, AI 생태계는 빠르게 진화하고 있습니다. 이번 글에서는 최신 AI 트렌드를 심층적으로 분석하고, 이러한 발전이 미래 기술 환경에 어떤 영향을 미칠지 살펴보겠습니다.
NVIDIA GTC, Baidu reasoning models and Gemini AI image generation
이 팟캐스트는 NVIDIA GTC의 발표, Baidu의 새로운 모델, Chain-of-Thought 추론의 결함, Gemini AI 이미지 생성 등 다양한 AI 분야의 최신 동향을 다룹니다. 특히 NVIDIA의 **Groot N1** 로봇 모델과 합성 데이터 생
lilys.ai
NVIDIA GTC에서 공개된 로봇 기술의 혁신적 진보
NVIDIA의 연례 컨퍼런스인 GTC(GPU Technology Conference)는 항상 AI 및 컴퓨팅 분야의 중요한 발표의 장이 되어왔습니다. 올해 컨퍼런스에서는 특히 로봇 기술에 관한 혁신적인 발표들이 큰 주목을 받았습니다.
Groot N1: 휴머노이드 로봇을 위한 혁신적 기반 모델
NVIDIA가 발표한 Groot N1은 휴머노이드 로봇을 위한 일반화 모델로, 인공지능의 인간 인지 과정에 영감을 받은 이중 시스템 구조를 채택했습니다. 이 모델은 인간의 사고 과정과 유사하게 직관적인 '시스템 1'과 분석적인 '시스템 2'를 결합하여 로봇이 더 자연스럽고 효율적으로 작동할 수 있도록 설계되었습니다.
이 모델의 특별한 점은 합성 데이터와 실제 데이터를 모두 활용한 학습 방식입니다. 로봇이 실제 환경에서 마주할 수 있는 다양한 상황을 시뮬레이션함으로써, 실제 배치 전에 발생할 수 있는 문제점들을 예측하고 해결할 수 있게 되었습니다.
Newton Physics Engine: 물리 시뮬레이션의 새로운 지평
로봇 개발에서 가장, 어려운 문제 중 하나는 실제 물리 환경을 정확하게 시뮬레이션하는 것입니다. NVIDIA의 Newton Physics Engine은 바로 이 문제를 해결하기 위해 개발되었으며, 고성능 GPU 가속 시뮬레이션을 통해 로봇의 움직임과 상호작용을 보다 정밀하게 예측할 수 있게 해줍니다.
"로봇이 복잡한 환경에서 미세한 움직임으로도 예기치 않은 행동을 할 수 있으므로, 안전 문제를 사전에 시뮬레이션하여 이해하는 것이 중요합니다."
이 엔진은 로봇 개발 주기를 획기적으로 단축시킬 뿐만 아니라, 더 안전하고 효율적인 로봇 시스템을 구축할 수 있는 기반을 마련합니다.
합성 데이터 생성: 로봇 학습의 게임 체인저
NVIDIA가 발표한 또 다른 혁신적인 기술은 합성 데이터 생성 프레임워크입니다. 로봇 학습에 있어 가장 큰 장벽 중 하나는 양질의 훈련 데이터를 충분히 확보하는 것인데, 합성 데이터 생성 기술은 이 문제를 해결하는 데 큰 도움이 됩니다.
이 프레임워크는 다양한 환경과 시나리오를 가상으로 생성하여 로봇이 실제 환경에 배치되기 전에 다양한 상황에 대응하는 방법을 학습할 수 있게 합니다. 특히 위험하거나 희귀한 상황에서의 대처 능력을 향상시키는 데 크게 기여할 것으로 기대됩니다.
NVIDIA의 시장 우위와 협업 전략
NVIDIA의 로봇 공학 관련 작업과 최근 발표된 Blackwell Dynamo의 성능 지표는 경쟁사들이 쉽게 따라잡기 힘든 수준입니다. 특히 DeepMind 및 Disney와의 전략적 협업은 NVIDIA가 AI 및 로봇 기술 분야에서 자신의 위치를 더욱 강화하고 있음을 보여줍니다.
이러한 협업은 실제 환경에서의 테스트와 훈련을 더욱 현실감 있게 진행할 수 있도록 도와주며, NVIDIA의 기술적 우위를 한층 더 공고히 하고 있습니다.
AI 접근성 확대를 위한 노력
NVIDIA는 단순히 기술 개발에만 집중하는 것이 아니라, 그 기술에 대한 접근성을 높이는 데도 노력을 기울이고 있습니다. DGX Spark와 같은 장치는 개발자들이 사이드 프로젝트나 혁신적인 아이디어를 실현할 수 있도록 지원하며, 교육 시장에서도 AI 학습 기회를 확대하는 데 기여할 것으로 보입니다.
이는 과거 Apple이 학교 시장에 성공적으로 진입했던 전략과 유사한 접근법으로, AI 기술의 대중화와 인재 육성에 긍정적인 영향을 미칠 것으로 예상됩니다.
Baidu의 AI 모델과 폐쇄형 소스 전략의 딜레마
중국의 대표적인 기술 기업인 Baidu는 최근 ERNIE X1과 ERNIE 4.5라는 두 개의 새로운 AI 모델을 발표했습니다. 특히 ERNIE X1은 DeepSeek의 모델과 경쟁할 것으로 보입니다. 그러나 주목할 만한 점은 Baidu가 이러한 모델들을 폐쇄형(클로즈드 소스) 방식으로 제공하기로 했다는 것입니다.
Baidu의 새로운 AI 모델과 시장 전략
Baidu는 중국에서 Google과 유사한 입지를 가진 검색 엔진 기업으로, AI 기술 개발에도 적극적으로 나서고 있습니다. ERNIE(Enhanced Representation through kNowledge IntEgration) 시리즈는 Baidu의 주력 AI 모델로, 언어 표현을 정보 있는 엔티티로 향상시키는 기술을 적용하고 있습니다4.
가격 책정 전략에서도 Baidu는 경쟁 모델의 절반 수준으로 설정하여 사용자 확보에 주력하고 있으며, 이는 시장에서의 입지를 강화하는 데 도움이 될 것으로 보입니다.
폐쇄형 소스 전략의 장단점
Baidu가 폐쇄형 소스 전략을 고수하는 이유는 무엇일까요? 기업으로서 Baidu는 데이터 프라이버시를 중시하며, 자사의 검색 엔진과 지도 서비스 등 다양한 AI 애플리케이션 간의 시너지를 극대화하기 위한 전략적 선택으로 보입니다.
그러나 최근 AI 업계의 트렌드는 오픈 소스 모델 쪽으로 기울고 있습니다. 오픈 소스 모델은 개발자 커뮤니티의 지원을 받아 빠르게 개선될 수 있고, 더 넓은 생태계를 구축할 수 있다는 장점이 있습니다.
ERNIE-4.0 모델은 학습 단계에서 코드 데이터를 활용했을 때 성능이 크게 향상되었으며, 특히 Macro F1 점수가 29.1 포인트, Micro F-점수가 33.7 포인트 향상되는 인상적인 결과를 보였습니다3. 이러한 성과는 오픈 소스 커뮤니티와의 협력을 통해 더욱 발전할 가능성이 있습니다.
미래의 오픈 소스 전환 가능성
전문가들은 Baidu가 결국 오픈 소스 전략으로 전환할 가능성이 높다고 전망합니다. 2023년 6월에 새로운 모델의 오픈 소스 계획을 발표했다는 점은 이러한 변화의 신호로 볼 수 있습니다.
오픈 소스 접근법은 모델의 투명성을 높이고, 시스템 테스트를 용이하게 하며, 더 많은 개발자를 유치하여 생태계를 확장하는 데 기여할 수 있습니다. 특히 OpenAI와 DeepSeek 같은 경쟁사들과의 경쟁에서 중요한 전략적 결정이 될 것입니다.
Chain-of-Thought 추론의 신뢰성 문제와 해결 방안
AI 모델의 추론 능력을 향상시키기 위한 방법론 중 하나인 Chain-of-Thought(COT)는 최근 많은 관심을 받고 있습니다. 그러나 이러한 추론 과정의 신뢰성에 대한 의문도 제기되고 있습니다.
Chain-of-Thought의 개념과 한계
Chain-of-Thought는 AI 모델이 문제를 해결할 때 여러 단계의 추론 과정을 거쳐 답변을 도출하는 방식입니다. 이 방식은 모델의 성능을 향상시키는 데 효과적인 것으로 알려져 있으나, 최근 연구에 따르면 이러한 추론 과정이 항상 신뢰할 수 있는 것은 아닙니다.
특히 모델의 추론 과정에는 인간의 인지 편향과 유사한 편향이 존재할 수 있으며, 이로 인해 잘못된 추론을 기반으로 결정을 내릴 가능성이 있습니다. 예를 들어, 동일한 질문이라도 문구에 따라 답변이 달라지는 현상이 관찰되었습니다.
최근 연구에서는 CRITIQUELLM이라는 모델을 통해 AI 응답에 대한 정보 있는 비평을 생성하고, 이를 통해 AI 모델의 평가를 개선하려는 시도가 이루어지고 있습니다1. 이러한 접근법은 Chain-of-Thought 추론의 품질을 높이는 데 기여할 수 있습니다.
추론 모델의 발전 방향
Chain-of-Thought는 모델의 결정 과정을 이해하는 데 유용한 도구로, 앞으로도 계속 발전할 것으로 예상됩니다. 현재 많은 기업들이 추론 모델을 개선하기 위해 Chain-of-Thought 프로세스 향상에 집중하고 있습니다.
이를 위해 자기 수정 모듈이나 구조화된 단계 검증과 같은 다양한 기술들이 도입되고 있으며, 이러한 기술들은 모델의 정확성을 향상시키고 Chain-of-Thought의 유용성을 유지하는 데 기여할 것입니다.
하이브리드 추론 프레임워크의 필요성
AI 모델의 신뢰성과 추론 능력을 향상시키기 위해서는 하이브리드 추론 프레임워크가 필수적입니다. 이러한 프레임워크는 다양한 추론 방식을 결합하여 더 강력하고 신뢰할 수 있는 AI 시스템을 구축하는 데 도움이 됩니다.
SLCoLM(Small Language model Collaboration with Large language Models)과 같은 협업 프레임워크는 작은 언어 모델과 대형 언어 모델을 결합하여 성능을 크게 향상시킬 수 있습니다. 실험 결과에 따르면, 이러한 접근법은 특히 특정 관계 카테고리에서 성능을 크게 개선하는 것으로 나타났습니다3.
Gemini 2.0의 세계 지식 통합과 이미지 생성 혁신
Google의 Gemini 2.0 Flash Experimental 모델은 베타 단계를 거쳐 이제 많은 사용자에게 공개되었습니다. 이 모델은 특히 이미지 생성 기능으로 주목받고 있으며, Google의 방대한 세계 지식을 활용하여 차별화된 경쟁력을 갖추고 있습니다.
Gemini의 이미지 생성 기술과 세계 지식의 결합
Gemini 모델은 Google의 지식 그래프와 연계하여 세계 지식을 이미지 생성에 통합했습니다. 이는 역사적 데이터와 사용자가 작성한 텍스트 및 이미지 데이터를 포함하는 광범위한 지식 기반을 활용하여 더 정확하고 맥락에 맞는 이미지를 생성할 수 있게 합니다.
Google의 이러한 접근법은 다른 경쟁 모델들과 차별화되는 점으로, 방대한 검색 데이터와 지식 그래프를 활용할 수 있는 Google만의 독특한 강점이라고 할 수 있습니다.
Google의 AI 경쟁력 회복
Google은 초기 AI 혁명에서 다소 뒤처진 것으로 평가받았지만, Gemini와 같은 인상적인 모델들의 출시를 통해 빠르게 경쟁력을 회복하고 있습니다. 특히 이미지 생성 분야에서 Google의 방대한 데이터 활용 능력은 중요한 경쟁 우위가 될 수 있습니다.
Gemini 2.0은 입력된 토큰 시퀀스(x1, x2, ..., xn)를 처리하여 다음 단어를 예측하는 아키텍처를 갖추고 있으며6, 이를 통해 사용자의 프롬프트에 맞는 이미지를 생성할 수 있습니다.
결론: AI 기술의 미래 전망과 윤리적 고려사항
NVIDIA의 로봇 기술 혁신, Baidu의 AI 모델 전략, Chain-of-Thought 추론의 신뢰성 문제, Gemini의 세계 지식 통합에 이르기까지, AI 기술은 다양한 방향으로 빠르게 발전하고 있습니다. 이러한 발전은 우리 사회와 산업에 큰 변화를 가져올 것으로 예상됩니다.
그러나 AI 기술이 발전함에 따라 윤리적 문제와 안전성 확보의 중요성도 더욱 부각되고 있습니다. 특히 Chain-of-Thought 추론의 신뢰성 문제는 AI 시스템의 결정이 실생활에 미치는 영향을 고려할 때 매우 중요한 문제입니다.
앞으로 AI 기술은 더욱 발전하여 우리 삶의 다양한 영역에 통합될 것이며, 이 과정에서 기술적 혁신과 함께 윤리적 고려사항을 균형 있게 다루는 것이 중요할 것입니다. 로봇 기술, 언어 모델, 이미지 생성 등 다양한 분야에서의 AI 혁신은, 안전하고 책임 있는 방식으로 사회에 도입될 때 비로소 진정한 가치를 발휘할 수 있을 것입니다.
여러분은 어떤 AI 기술의 발전이 가장 기대되나요? AI 기술의 윤리적 측면에 대해 어떤 생각을 가지고 계신가요? 아래 댓글로 여러분의 의견을 들려주세요!
태그
#AI기술 #NVIDIA #Baidu #ChainofThought #Gemini #로봇기술 #AIethics #GrootN1 #ERNIE #AIimage #기술혁신 #GoogleAI #AIsafety #AItrends #AImodel
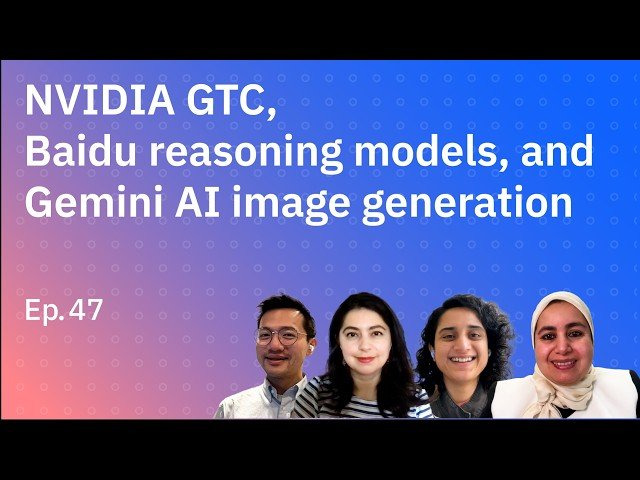
2025 AI Technology Outlook: From NVIDIA's Robot Revolution to Baidu's Closed Strategy
As the pace of AI technology development increases exponentially, exciting new opportunities are unfolding for developers and businesses. From innovative robot technology announced at NVIDIA GTC to Baidu's strategic AI model approach and the reliability issues of Chain-of-Thought reasoning, the AI ecosystem is rapidly evolving. In this article, we'll analyze the latest AI trends in depth and examine how these developments will affect the future technology landscape.
Innovative Advances in Robot Technology Unveiled at NVIDIA GTC
NVIDIA's annual conference, GTC (GPU Technology Conference), has always been a platform for important announcements in AI and computing. At this year's conference, innovative presentations on robot technology garnered significant attention.
Groot N1: Innovative Foundation Model for Humanoid Robots
NVIDIA's announced Groot N1 is a generalization model for humanoid robots that adopts a dual-system architecture inspired by human cognitive processes. This model combines an intuitive 'System 1' and an analytical 'System 2' similar to human thought processes, designed to make robots operate more naturally and efficiently.
What makes this model special is its learning approach that utilizes both synthetic and real data. By simulating various situations robots may encounter in real environments, it becomes possible to predict and solve problems that might occur before actual deployment.
Newton Physics Engine: New Horizons in Physical Simulation
One of the most challenging problems in robot development is accurately simulating the physical environment. NVIDIA's Newton Physics Engine was developed to address this issue, enabling more precise prediction of robot movements and interactions through high-performance GPU-accelerated simulation.
"Since robots can exhibit unexpected behaviors even with subtle movements in complex environments, it's important to understand safety issues by simulating them in advance."
This engine not only dramatically shortens the robot development cycle but also establishes a foundation for building safer and more efficient robot systems.
Synthetic Data Generation: Game Changer for Robot Learning
Another innovative technology announced by NVIDIA is the synthetic data generation framework. One of the biggest barriers to robot learning is securing sufficient quality training data, and synthetic data generation technology helps solve this problem.
This framework virtually generates various environments and scenarios, allowing robots to learn how to respond to diverse situations before being deployed in real environments. It is especially expected to contribute significantly to improving coping abilities in dangerous or rare situations.
NVIDIA's Market Advantage and Collaboration Strategy
The performance metrics of NVIDIA's robotics work and recently announced Blackwell Dynamo are at a level that competitors find difficult to match. In particular, strategic collaborations with DeepMind and Disney show that NVIDIA is further strengthening its position in AI and robotics technology.
These collaborations help make testing and training in real environments more realistic, further solidifying NVIDIA's technological edge.
Efforts to Expand AI Accessibility
NVIDIA is not just focusing on technology development but also striving to increase accessibility to that technology. Devices like DGX Spark support developers in realizing side projects or innovative ideas and are expected to contribute to expanding AI learning opportunities in the education market as well.
This is a similar approach to Apple's successful entry into the school market in the past and is expected to have a positive impact on the popularization of AI technology and talent development.
The Dilemma of Baidu's AI Models and Closed Source Strategy
Baidu, a leading technology company in China, recently announced two new AI models: ERNIE X1 and ERNIE 4.5. ERNIE X1, in particular, is expected to compete with DeepSeek's model. However, what's notable is that Baidu has decided to provide these models in a closed source manner.
Baidu's New AI Models and Market Strategy
Baidu is a search engine company with a position similar to Google in China and is actively engaging in AI technology development. The ERNIE (Enhanced Representation through kNowledge IntEgration) series is Baidu's flagship AI model, applying technology that enhances language representation with informative entities4.
In pricing strategy, Baidu is focusing on securing users by setting prices at half the level of competing models, which is expected to help strengthen its position in the market.
Pros and Cons of Closed Source Strategy
Why is Baidu sticking to a closed source strategy? As a company, Baidu values data privacy and appears to be making a strategic choice to maximize synergy between various AI applications such as its search engine and map services.
However, the recent trend in the AI industry is leaning toward open source models. Open source models have the advantage of being rapidly improved with the support of the developer community and building a broader ecosystem.
The ERNIE-4.0 model showed significantly improved performance when utilizing code data in the learning phase, with impressive results including a 29.1-point increase in Macro F1 score and a 33.7-point increase in Micro F-score3. These achievements have the potential to develop further through collaboration with the open source community.
Possibility of Future Transition to Open Source
Experts predict that Baidu is likely to transition to an open source strategy eventually. The announcement of plans to open source a new model in June 2023 can be seen as a signal of this change.
An open source approach can enhance model transparency, facilitate system testing, and contribute to expanding the ecosystem by attracting more developers. This will be an important strategic decision especially in competition with rivals like OpenAI and DeepSeek.
Reliability Issues and Solutions for Chain-of-Thought Reasoning
Chain-of-Thought (COT), one of the methodologies for improving the reasoning ability of AI models, has been receiving a lot of attention recently. However, questions are also being raised about the reliability of this reasoning process.
Concept and Limitations of Chain-of-Thought
Chain-of-Thought is a method where AI models derive answers through multiple stages of reasoning when solving problems. While this method is known to be effective in improving model performance, recent studies suggest that these reasoning processes are not always reliable.
In particular, biases similar to human cognitive biases may exist in a model's reasoning process, potentially leading to decisions based on incorrect reasoning. For example, the phenomenon of different answers depending on phrasing has been observed even for the same question.
Recent research attempts to improve AI model evaluation through a model called CRITIQUELLM, which generates informative critiques of AI responses1. This approach can contribute to improving the quality of Chain-of-Thought reasoning.
Development Direction of Reasoning Models
Chain-of-Thought is a useful tool for understanding a model's decision process and is expected to continue developing. Currently, many companies are focusing on improving the Chain-of-Thought process to enhance reasoning models.
Various techniques such as self-correction modules and structured step verification are being introduced for this purpose, which will contribute to improving model accuracy and maintaining the usefulness of Chain-of-Thought.
Need for Hybrid Reasoning Frameworks
Hybrid reasoning frameworks are essential for improving the reliability and reasoning ability of AI models. Such frameworks help build more powerful and reliable AI systems by combining various reasoning methods.
Collaboration frameworks like SLCoLM (Small Language model Collaboration with Large language Models) can significantly improve performance by combining small and large language models. According to experimental results, this approach shows significant performance improvements especially in certain relationship categories3.
Gemini 2.0's World Knowledge Integration and Image Generation Innovation
Google's Gemini 2.0 Flash Experimental model has been released to many users after going through a beta phase. This model is particularly notable for its image generation capabilities and has differentiated competitiveness by leveraging Google's vast world knowledge.
Combination of Gemini's Image Generation Technology and World Knowledge
The Gemini model integrates world knowledge into image generation in conjunction with Google's knowledge graph. This enables the generation of more accurate and contextually appropriate images by utilizing an extensive knowledge base that includes historical data and user-created text and image data.
Google's approach is a point of differentiation from other competing models and a unique strength of Google that can leverage extensive search data and knowledge graphs.
Google's Recovery of AI Competitiveness
Although Google was evaluated as somewhat behind in the early AI revolution, it is quickly recovering competitiveness through the release of impressive models like Gemini. Particularly in the field of image generation, Google's vast data utilization capability can be an important competitive advantage.
Gemini 2.0 is equipped with an architecture that processes input token sequences (x1, x2, ..., xn) to predict the next word6, allowing it to generate images that match user prompts.
Conclusion: Future Outlook and Ethical Considerations for AI Technology
From NVIDIA's robot technology innovation to Baidu's AI model strategy, reliability issues in Chain-of-Thought reasoning, and Gemini's world knowledge integration, AI technology is rapidly developing in various directions. These developments are expected to bring significant changes to our society and industries.
However, as AI technology advances, the importance of ethical issues and ensuring safety is also becoming more prominent. In particular, the reliability issue of Chain-of-Thought reasoning is a very important issue when considering the impact of AI system decisions on real life.
In the future, AI technology will further develop and integrate into various areas of our lives, and in this process, it will be important to balance technological innovation with ethical considerations. AI innovations in various fields such as robotics, language models, and image generation can only demonstrate true value when they are introduced to society in a safe and responsible manner.
Which AI technology development are you most looking forward to? What thoughts do you have about the ethical aspects of AI technology? Please share your opinions in the comments below!
Tags
#AITechnology #NVIDIA #Baidu #ChainofThought #Gemini #RobotTechnology #AIEthics #GrootN1 #ERNIE #AIImage #TechInnovation #GoogleAI #AISafety #AITrends #AIModel
Citations:
- https://openreview.net/attachment?id=ZBZEZZsINa&name=pdf
- https://arxiv.org/pdf/2311.18702.pdf
- https://arxiv.org/pdf/2402.14373.pdf
- https://arxiv.org/pdf/2404.12014.pdf
- https://openreview.net/pdf?id=KIPJKST4gw
- https://arxiv.org/pdf/2402.06853.pdf
- https://openreview.net/pdf?id=ViZcgDQjyG
- https://arxiv.org/pdf/2112.12731.pdf
- http://arxiv.org/pdf/2311.12351.pdf
- https://openreview.net/attachment?id=flU8ghMEI4&name=pdf
- https://arxiv.org/pdf/2411.17679.pdf
- https://arxiv.org/pdf/1808.01478.pdf
- http://arxiv.org/pdf/2406.13843.pdf
'DeepResearch' 카테고리의 다른 글
텐센트의 T1 AI 모델: 중국 AI 혁명의 새로운 챔피언이 될 수 있을까? (0) | 2025.03.24 |
---|---|
업무 혁명: 생성형 AI로 당신의 생산성을 2배로 높이는 실전 가이드 (0) | 2025.03.23 |
2025 AI 혁신의 최전선: NVIDIA, Baidu, Gemini 기술로 바라본 미래 (1) | 2025.03.23 |
AI와 인간 혁신의 미래: Google DeepMind의 통찰로 바라본 협력의 시대 (0) | 2025.03.22 |
🚀 AI 혁명의 최전선: 소프트뱅크의 앰페어 인수와 기술 산업의 새로운 지평 (0) | 2025.03.22 |