인공지능 기술이 폭발적으로 발전하는 2025년, 로봇 기술부터 생성형 AI까지 다양한 혁신들이 우리 삶을 빠르게 변화시키고 있습니다. NVIDIA의 혁신적인 로봇 기반 모델, Baidu의 새로운 언어 모델 전략, Google의 Gemini 이미지 생성까지 - 이번 포스트에서는 최신 AI 기술 동향과 그 의미를 심층적으로 살펴봅니다.
A Practical Quantum Computer Is Coming! But When?
이 영상은 **양자 컴퓨터**의 현재 개발 상황과 미래 전망에 대해 심층적으로 다룹니다. 양자 컴퓨터는 기존 컴퓨터로는 풀 수 없는 복잡한 문제를 해결할 잠재력을 가지고 있으며, 재료 과학, 제
lilys.ai
NVIDIA GTC의 로봇 기술 혁신, 인간의 삶을 바꾸다
NVIDIA의 최근 GTC(GPU Technology Conference)는 AI와 로봇 기술의 미래를 엿볼 수 있는 중요한 행사였습니다. 특히 주목받은 것은 Groot N1 모델로, 인간형 로봇을 위한 혁신적인 기반 모델입니다.
Groot N1: 인간형 로봇의 미래
Groot N1은 일반적인 목적의 로봇을 위한 오픈 파운데이션 모델로, 인간 세계에서 범용적인 자율성을 구축하기 위한 플랫폼으로 주목받고 있습니다. 이 모델의 가장 큰 특징은 이중 시스템 아키텍처입니다.
"일반 목적 로봇에는 다재다능한 몸체와 지능적인 마인드가 필요합니다. 최근 인간형 로봇의 발전은 인간 세계에서 범용적 자율성을 구축하기 위한 하드웨어 플랫폼으로 큰 가능성을 보여주고 있습니다."13
Groot N1은 비전-언어-액션(VLA) 모델로, 다음과 같은 구조를 가집니다:
- 시스템 2: 시각과 언어 지침을 통해 환경을 해석하는 비전-언어 모듈
- 시스템 1: 실시간으로 유동적인 모터 액션을 생성하는 확산 트랜스포머 모듈
이 두 모듈은 긴밀하게 연결되어 있으며, 함께 엔드투엔드로 훈련됩니다. 특별히 주목할 점은 Groot N1이 실제 로봇 궤적, 인간 비디오, 합성 생성 데이터셋 등의 이질적인 데이터 혼합으로 훈련된다는 점입니다13.
합성 데이터와 시뮬레이션: 로봇 훈련의 혁명
NVIDIA는 단순히 로봇 모델을 개발하는 것에 그치지 않고, 로봇 시뮬레이션을 위한 Newton Physics Engine과 합성 데이터 생성 프레임워크를 함께 발표했습니다. 이는 로봇 개발의 패러다임을 바꾸는 중요한 요소입니다.
로봇 시뮬레이션은 NVIDIA Omniverse Isaac Sim에서 진행되며, 그래스프 샘플링과 충돌 체크 작업은 NVIDIA Warp를 사용하여 GPU로 가속화됩니다1. 이러한 가상 환경에서의 테스트는 로봇을 실제 환경에 배치하기 전에 안전성과 성능을 확보하는 데 핵심적입니다.
"로봇 성능을 높이기 위해서는 물리 엔진과 RAP 프레임워크를 활용한 고성능 GPU 가속 시뮬레이션이 필수적입니다. 실제 환경에 배치하기 전에 가상 환경에서 로봇 시스템을 훈련하고 테스트할 수 있는 능력은 매우 중요한 발전입니다."
합성 데이터 생성의 중요성은 아무리 강조해도 지나치지 않습니다. 로봇을 훈련할 때 직면하는 가장 큰 문제 중 하나는 데이터의 부족입니다. 특히 다양한 환경과 조건에서의 테스트 데이터는 매우 제한적입니다. NVIDIA의 합성 데이터 생성 프레임워크는 이 문제를 해결하며, 로봇이 실제 환경에서 마주할 수 있는 무수한 시나리오를 시뮬레이션할 수 있게 해줍니다19.
NVIDIA의 시장 우위와 협업 생태계
NVIDIA는 DeepMind 및 Disney와 같은 주요 기업들과의 협업을 통해 로봇 기술을 더욱 발전시키고 있습니다. 이러한 파트너십은 NVIDIA의 시장 우위를 강화하는 동시에, 로봇 기술의 실용적 응용 사례를 확대하고 있습니다.
"NVIDIA의 로봇 공학 작업과 Blackwell Dynamo에 대한 성능 지표가 매우 뛰어나며, 이는 경쟁자들이 이들을 따라잡기 어렵게 만듭니다. 시장에서 NVIDIA와 비슷한 성과를 달성하기는 힘들며, 그들의 생태계가 큰 격차를 만들어 내고 있습니다."
특히 주목할 만한 점은 NVIDIA가 이러한 혁신 기술들을 오픈 소스로 제공한다는 사실입니다. 이는 개발자 커뮤니티의 접근성을 높이고, 더 많은 혁신을 촉진하는 전략적 결정으로 볼 수 있습니다.
또한 최근 발표된 DGX Spark와 같은 새로운 장치는 연구자들에게 더 많은 실험 기회를 제공하며, AI 기술의 민주화에 기여할 것으로 예상됩니다. 이는 교육 시장에서도 큰 영향을 미칠 것으로 보이며, 과거 Apple이 학교 시장에 진입했던 것과 유사한 패턴을 보일 것으로 예측됩니다.
Baidu의 새로운 AI 모델과 오픈 소스 딜레마
중국의 기술 거인 Baidu는 최근 ERNIE X1과 ERNIE 4.5 두 개의 새로운 AI 모델을 발표했습니다. 특히 ERNIE X1은 중국의 오픈 소스 모델인 DeepSeek와 경쟁하기 위해 개발되었습니다. 하지만 이 두 모델 모두 '클로즈드 소스'로 제공된다는 점이 주목할 만합니다.
ERNIE의 혁신과 전략적 위치
2019년에 처음 소개된 ERNIE(Enhanced Representation through kNowledge IntEgration)는 지식 통합을 통한 향상된 표현을 목표로 개발되었습니다. BERT의 마스킹 전략에서 영감을 받아, 엔티티 수준 마스킹과 구문 수준 마스킹을 포함한 지식 마스킹 전략을 사용합니다5.
"ERNIE는 지식 마스킹 전략을 통해 언어 표현을 학습하도록 설계되었으며, 여기에는 엔티티 수준 마스킹과 구문 수준 마스킹이 포함됩니다. 엔티티 수준 전략은 일반적으로 여러 단어로 구성된 엔티티를 마스킹하고, 구문 수준 전략은 개념적 단위로서 함께 서있는 여러 단어로 구성된 전체 구문을 마스킹합니다."5
ERNIE는 자연어 추론, 의미 유사성, 개체명 인식, 감정 분석, 질문 응답 등 5가지 중국어 자연어 처리 작업에서 새로운 최고 성능을 달성했습니다. 또한 ERNIE의 데이터셋은 중국 위키피디아, Baidu Baike, Baidu 뉴스, Baidu Tieba 등 다양한 소스를 포함합니다5.
최근 발표된 모델들의 성능은 매우 인상적입니다. 예를 들어, ERNIE-4.0(ICL+SLCoLM)은 다양한 NLP 작업에서 상당한 성능 향상을 보여주고 있습니다4.
클로즈드 소스 vs 오픈 소스: Baidu의 선택
Baidu가 ERNIE X1과 ERNIE 4.5를 클로즈드 소스로 유지하는 결정은 현재 AI 산업의 트렌드와는 조금 다릅니다. 많은 주요 AI 기업들이 적어도 부분적으로는 모델을 오픈 소스화하는 경향이 있는데, Baidu는 왜 다른 전략을 선택했을까요?
이는 Baidu의 데이터 프라이버시에 대한 장기적인 철학과 관련이 있을 수 있습니다. Baidu는 중국의 Google과 같은 위치에 있는 검색 엔진 기업으로, 이미 대규모 사용자 기반을 보유하고 있습니다. 이러한 기업들은 가끔 오픈소스 전략보다는 기존의 사용자 기반과 서비스를 활용하는 전략을 선호합니다.
그러나 전문가들은 Baidu도 결국 오픈 소스 전략을 수용할 필요가 있을 것이라고 예측합니다. 오픈 소스 모델은 개발자 커뮤니티의 기여를 통해 더 빠르게 개선될 수 있으며, 더 넓은 생태계를 구축하는 데 도움이 됩니다.
"오픈 소스 전략은 더 많은 개발자를 유치하고, 생태계를 구축하며, 사용자 채택을 촉진하는 데 필수적입니다."
또한 Baidu는 경쟁력을 유지하기 위해 가격 전략도 활용하고 있습니다. 그들은 새로운 모델의 가격을 경쟁 모델의 절반으로 설정하여 사용자를 유치하려고 합니다. 이는 시장 점유율을 확보하기 위한 적극적인 전략으로 볼 수 있습니다.
Chain-of-Thought 추론의 신뢰성 문제
최근 AI 연구에서 Chain-of-Thought(CoT) 추론 방식이 큰 주목을 받고 있습니다. 이 방식은 AI 모델이 단계별로 문제를 해결해 나가는 과정을 보여주어 복잡한 추론 작업에서 성능을 향상시키는 것으로 알려져 있습니다.
Chain-of-Thought의 한계와 도전
CoT 접근법은 추론 성능과 상호 운용성을 향상시켰지만, 여전히 두 가지 주요 도전 과제가 있습니다9:
- 분해할 수 없는 문제: 모든 문제가 단계별로 분해될 수 있는 것은 아닙니다.
- 오류 가능성: 특히 작은 규모의 언어 모델에서는 오류가 있는 추론 체인이 발생할 수 있습니다.
이러한 한계를 해결하기 위해 '선택적 필터링 추론기(SelF-Reasoner)'와 같은 새로운 접근법이 제안되고 있습니다. 이 접근법은 질문과 후보 추론 체인 사이의 함의 관계를 평가하여, 추론 체인이 신뢰할 만할 때는 CoT 추론을 진행하고, 그렇지 않을 때는 직접 답변을 예측하는 방식입니다9.
더 심각한 문제는 CoT 추론이 **불충실(unfaithful)**할 수 있다는 점입니다. 즉, 제시된 추론 과정이 모델의 실제 내부 동작을 정확하게 반영하지 않을 수 있습니다6. 이는 특히 의료와 같은 중요한 분야에서 AI 모델을 사용할 때 큰 문제가 될 수 있습니다.
"대규모 언어 모델(LLM)이 헬스케어와 같은 중요한 도메인에서 점점 더 많이 사용됨에 따라, 이러한 모델들을 신뢰할 수 있게 만드는 것이 필수적입니다. 이를 위해 CoT(Chain-of-Thought) 프롬프팅이 LLM의 잠재적 투명성 소스로 등장했습니다. CoT 추론은 인간에게 매력적이지만, 이전 연구들은 이러한 추론 체인이 충실하지 않다는 것을 보여주었습니다."6
CoT 신뢰성 향상을 위한 노력
CoT 추론의 신뢰성 문제를 해결하기 위한 다양한 접근법이 연구되고 있습니다:
- 자연 프로그램(Natural Program): 자연어 기반 연역적 추론 형식으로, LLM이 추론 체인의 자체 검증을 수행할 수 있게 합니다7.
- CoT Rerailer: 복잡한 추론 작업에서 오류 감지 및 수정을 통해 LLM의 신뢰성을 향상시키는 방법입니다. 이 접근법은 자체 일관성과 다중 에이전트 토론 시스템을 사용하여 추론 과정에서 오류를 식별하고 수정합니다8.
- Chain of Preference Optimization(CPO): ToT(Tree-of-Thought) 방법으로 구성된 검색 트리를 활용하여 LLM을 미세 조정함으로써, CoT가 유사하거나 더 나은 성능을 달성할 수 있게 합니다10.
"CPO는 질문 응답, 사실 검증, 산술 추론 등 다양한 복잡한 문제를 해결하는 데 있어 LLM 성능을 크게 향상시킵니다."10
그러나 이러한 개선에도 불구하고, CoT 추론의 근본적인 신뢰성 문제는 여전히 해결해야 할 과제로 남아 있습니다. 최근 연구에 따르면, 인컨텍스트 학습, 미세 조정, 활성화 편집과 같은 일반적인 전략들이 CoT 추론의 충실도를 향상시키는 데 제한된 성공을 거두었다고 합니다6.
더 우려되는 점은 H-CoT(Hijacking Chain-of-Thought)와 같은 기법이 등장하여 모델의 안전 추론 메커니즘을 우회할 수 있다는 것입니다. 이 공격 방법은 모델 자체의 중간 추론을 활용하여 그 안전 추론 메커니즘을 해킹합니다16.
Gemini 2.0의 세계 지식과 물리적 이해
Google의 Gemini 2.0은 이제 베타 단계를 넘어 많은 사용자에게 공개되었습니다. 특히 주목할 만한 기능은 이미지 생성 능력입니다. Google은 Gemini가 세계 지식을 통합하여 뛰어난 이미지 생성 성능을 달성했다고 주장합니다.
Gemini의 물리적 세계 이해
흥미로운 점은 Gemini가 단순한 텍스트나 이미지 생성을 넘어 물리적 세계의 현상까지 이해하고 있다는 것입니다. 최근 연구에 따르면, Gemini 모델은 소셜 미디어 게시물과 같은 비정형 소스에서 지진 지반 진동 강도를 추정할 수 있습니다11.
"이 논문은 소셜 미디어 게시물과 같은 비관습적 소스에서 지구의 물리적 현상에 대한 과학적으로 가치 있는 정보를 추출하는 새로운 접근법을 제시합니다. 최첨단 대규모 언어 모델(LLM)인 Gemini 1.5 Pro를 사용하여 이러한 비구조화된 게시물에서 지진 지반 진동 강도를 추정합니다."11
Gemini는 인터넷에서 훈련된 데이터를 통해 물리적 현상에 대한 고유한 이해를 발전시켰을 가능성이 있습니다. 특히, Google의 Gemini 모델은 지진 규모, 거리, MMI 강도 사이의 일반적인 관계에 대한 단순화된 이해를 보여주며, 이는 확립된 모델과 동일하지는 않지만 관측 데이터를 정확하게 설명합니다11.
Gemini 2.0의 성별 및 콘텐츠 편향
Gemini 2.0 Flash Experimental에 대한 최근 연구는 콘텐츠 조절과 성별 격차에 초점을 맞추고 있습니다. 이 연구는 Gemini 2.0이 ChatGPT-4o에 비해 성별 편향이 감소했음을 보여줍니다. 특히 여성 특화 프롬프트의 수용률이 ChatGPT-4o보다 상당히 증가했습니다14.
"Gemini 2.0은 성별 편향이 감소했으며, 특히 여성 특화 프롬프트의 수용률이 ChatGPT-4o에서 얻은 결과에 비해 크게 증가했습니다."14
그러나 이러한 변화가 개선인지는 논쟁의 여지가 있습니다. 성별 편향이 감소했지만, 이는 남성과 여성 모두에 대한 폭력적 콘텐츠를 더 많이 허용하는 대가로 이루어졌으며, 이는 폭력을 완화하기보다 정상화할 수 있습니다. 또한 남성 특화 프롬프트는 여전히 여성 특화 프롬프트보다 일반적으로 더 높은 수용률을 보입니다14.
이러한 발견은 윤리적 표준과 AI 시스템의 정렬 복잡성을 강조하며, 특정 편향을 줄이는 데 진전이 있지만 모델의 허용성의 더 넓은 영향에 대한 우려를 제기합니다.
결론: AI 기술의 미래와 윤리적 과제
우리는 AI 기술의 빠른 발전을 목격하고 있습니다. NVIDIA의 로봇 기술, Baidu의 언어 모델, Google의 Gemini 등 다양한 혁신이 등장하고 있습니다. 그러나 이러한 발전에는 여러 과제와 우려가 따릅니다.
특히 Chain-of-Thought 추론의 신뢰성 문제는 AI 기술이 중요한 의사결정 영역으로 확장됨에 따라 더욱 중요해질 것입니다. 또한 AI 모델의 편향과 안전성 문제도 계속해서 연구되고 개선되어야 할 부분입니다.
AI 기술이 계속해서 발전함에 따라, 우리는 이러한 기술의 혜택을 극대화하면서도 잠재적인 위험을 최소화하는 방법을 찾아야 합니다. 이는 기술적인 개선뿐만 아니라, 윤리적 가이드라인과 규제 프레임워크의 발전을 필요로 합니다.
AI 기술은 이제 단순한 도구를 넘어, 우리 사회의 핵심 인프라로 자리 잡아가고 있습니다. 이러한 변화 속에서 기술의 발전과 윤리적 고려 사이의 균형을 찾는 것이 우리 모두의 과제입니다.
여러분은 이러한 AI 기술의 발전에 어떤 기대를 갖고 계신가요? 로봇 기술의 발전이 우리 일상에 어떤 변화를 가져올 것이라고 생각하시나요? 댓글로 여러분의 생각을 공유해주세요!
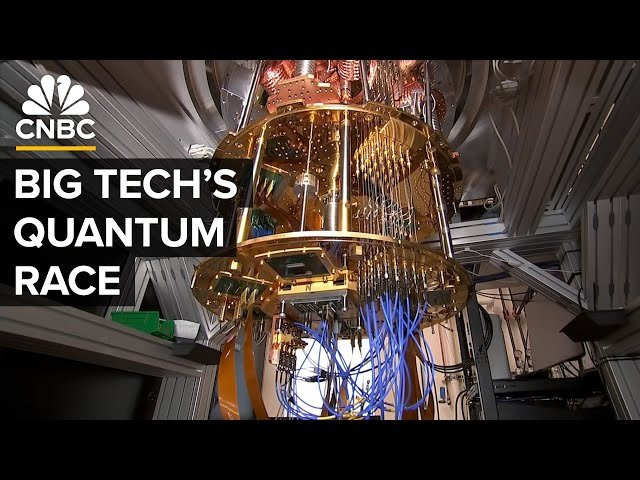
The Frontiers of AI Innovation in 2025: The Future Shaped by NVIDIA, Baidu, and Gemini Technologies
In 2025, with artificial intelligence technology exploding in development, various innovations from robotics to generative AI are rapidly changing our lives. NVIDIA's innovative robot foundation models, Baidu's new language model strategy, and Google's Gemini image generation - in this post, we delve deeply into the latest AI technology trends and their significance.
NVIDIA GTC's Robotics Innovation Transforming Human Life
NVIDIA's recent GTC (GPU Technology Conference) was a significant event offering a glimpse into the future of AI and robotics technology. Particularly noteworthy was the Groot N1 model, an innovative foundation model for humanoid robots.
Groot N1: The Future of Humanoid Robots
Groot N1 is an open foundation model for general-purpose robots, gaining attention as a platform for building generalist autonomy in the human world. The most distinctive feature of this model is its dual-system architecture.
"General-purpose robots need a versatile body and an intelligent mind. Recent advancements in humanoid robots have shown great promise as a hardware platform for building generalist autonomy in the human world."13
Groot N1 is a Vision-Language-Action (VLA) model with the following structure:
- System 2: A vision-language module that interprets the environment through vision and language instructions
- System 1: A diffusion transformer module that generates fluid motor actions in real time
These two modules are tightly coupled and jointly trained end-to-end. A particularly noteworthy point is that Groot N1 is trained with a heterogeneous mixture of real-robot trajectories, human videos, and synthetically generated datasets13.
Synthetic Data and Simulation: The Revolution in Robot Training
NVIDIA has not only developed robot models but also announced the Newton Physics Engine for robot simulation and a synthetic data generation framework. These are important elements changing the paradigm of robot development.
Robot simulation is conducted in NVIDIA Omniverse Isaac Sim, with grasp sampling and collision checking operations GPU-accelerated using NVIDIA Warp1. Such testing in virtual environments is crucial for ensuring safety and performance before deploying robots in real environments.
"For improved robot performance, high-performance GPU-accelerated simulation utilizing physics engines and RAP frameworks is essential. The ability to train and test robot systems in virtual environments before deployment in real environments is a very important advancement."
The importance of synthetic data generation cannot be overstated. One of the biggest problems when training robots is the lack of data. Test data in various environments and conditions is particularly limited. NVIDIA's synthetic data generation framework solves this problem, allowing simulation of countless scenarios that robots could encounter in real environments19.
NVIDIA's Market Advantage and Collaborative Ecosystem
NVIDIA is further advancing robotics technology through collaborations with major companies like DeepMind and Disney. These partnerships strengthen NVIDIA's market position while expanding practical applications of robotics technology.
"NVIDIA's robotics work and performance metrics for Blackwell Dynamo are outstanding, making it difficult for competitors to catch up. It's hard to achieve similar results to NVIDIA in the market, and their ecosystem is creating a significant gap."
Particularly noteworthy is that NVIDIA provides these innovative technologies as open source. This can be seen as a strategic decision to increase accessibility for the developer community and promote more innovation.
Additionally, new devices like DGX Spark recently announced are expected to provide researchers with more experimental opportunities and contribute to the democratization of AI technology. This is also expected to have a significant impact on the education market, showing a pattern similar to Apple's entry into the school market in the past.
Baidu's New AI Models and the Open Source Dilemma
Chinese tech giant Baidu recently announced two new AI models, ERNIE X1 and ERNIE 4.5. ERNIE X1, in particular, was developed to compete with DeepSeek, an open-source model from China. However, it's notable that both models are provided as 'closed source'.
ERNIE's Innovation and Strategic Position
First introduced in 2019, ERNIE (Enhanced Representation through kNowledge IntEgration) was developed with the goal of enhanced representation through knowledge integration. Inspired by BERT's masking strategy, it uses knowledge masking strategies including entity-level masking and phrase-level masking5.
"ERNIE is designed to learn language representation enhanced by knowledge masking strategies, which includes entity-level masking and phrase-level masking. Entity-level strategy masks entities which are usually composed of multiple words. Phrase-level strategy masks the whole phrase which is composed of several words standing together as a conceptual unit."5
ERNIE has achieved new state-of-the-art results on five Chinese natural language processing tasks including natural language inference, semantic similarity, named entity recognition, sentiment analysis, and question answering. Additionally, ERNIE's dataset includes various sources such as Chinese Wikipedia, Baidu Baike, Baidu news, and Baidu Tieba5.
The performance of recently announced models is very impressive. For example, ERNIE-4.0(ICL+SLCoLM) shows significant performance improvements in various NLP tasks4.
Closed Source vs Open Source: Baidu's Choice
Baidu's decision to keep ERNIE X1 and ERNIE 4.5 closed source is somewhat different from the current trend in the AI industry. While many major AI companies tend to open-source their models at least partially, why did Baidu choose a different strategy?
This may be related to Baidu's long-term philosophy regarding data privacy. Baidu is a search engine company positioned like Google in China, already having a large user base. Such companies sometimes prefer strategies that leverage their existing user base and services rather than open-source strategies.
However, experts predict that Baidu will also need to adopt an open-source strategy eventually. Open-source models can be improved more quickly through contributions from the developer community and help build a broader ecosystem.
"Open-source strategy is essential for attracting more developers, building an ecosystem, and promoting user adoption."
Additionally, Baidu is also utilizing a pricing strategy to maintain competitiveness. They are setting the price of their new models at half that of competing models to attract users. This can be seen as an aggressive strategy to secure market share.
The Reliability Issue of Chain-of-Thought Reasoning
Chain-of-Thought (CoT) reasoning has been receiving significant attention in recent AI research. This approach is known to improve performance in complex reasoning tasks by showing the step-by-step problem-solving process of AI models.
Limitations and Challenges of Chain-of-Thought
While CoT approaches have shown improvements in reasoning performance and interoperability, there are still two main challenges9:
- Indecomposable questions: Not all problems can be decomposed step by step.
- Error potential: Erroneous reasoning chains can occur, particularly in small-scale language models.
To address these limitations, new approaches like 'selective filtering reasoner (SelF-Reasoner)' have been proposed. This approach assesses the entailment relationship between the question and the candidate reasoning chain, proceeding with CoT reasoning when the reasoning chain demonstrates confidence, and otherwise opting to predict the answer directly9.
A more serious issue is that CoT reasoning can be unfaithful. That is, the presented reasoning process may not accurately reflect the model's actual internal operation6. This can be a major problem especially when using AI models in critical areas such as healthcare.
"As Large Language Models (LLMs) are being increasingly employed in critical domains such as healthcare, it is essential to make these models trustworthy. In this pursuit, Chain-of-Thought (CoT) prompting has emerged as a potential source of transparency in LLMs. While CoT reasoning is appealing to humans, prior studies have shown that these reasoning chains are not faithful."6
Efforts to Improve CoT Reliability
Various approaches are being researched to address the reliability issues of CoT reasoning:
- Natural Program: A natural language-based deductive reasoning format that enables LLMs to carry out self-verification of reasoning chains7.
- CoT Rerailer: A method to enhance the reliability of LLMs in complex reasoning tasks through error detection and correction. This approach uses self-consistency and multi-agent debate systems to identify and rectify errors in the reasoning process8.
- Chain of Preference Optimization (CPO): Fine-tuning LLMs leveraging the search tree constructed by ToT (Tree-of-Thought) to allow CoT to achieve similar or better performance10.
"CPO significantly improves LLM performance in solving a variety of complex problems, including question answering, fact verification, and arithmetic reasoning."10
However, despite these improvements, the fundamental reliability issue of CoT reasoning remains a challenge to be solved. According to recent research, common strategies such as in-context learning, fine-tuning, and activation editing have had limited success in improving the faithfulness of CoT reasoning6.
More concerningly, techniques like H-CoT (Hijacking Chain-of-Thought) have emerged that can bypass the model's safety reasoning mechanism. This attack method leverages the model's own intermediate reasoning to hack its safety reasoning mechanism16.
Gemini 2.0's World Knowledge and Physical Understanding
Google's Gemini 2.0 has now moved beyond the beta stage and is available to many users. A particularly noteworthy feature is its image generation capability. Google claims that Gemini has achieved outstanding image generation performance by integrating world knowledge.
Gemini's Understanding of the Physical World
Interestingly, Gemini goes beyond simple text or image generation to understand phenomena in the physical world. According to recent research, Gemini models can estimate earthquake ground shaking intensity from unstructured sources such as social media posts11.
"This paper presents a novel approach to extract scientifically valuable information about Earth's physical phenomena from unconventional sources, such as multi-modal social media posts. Employing a state-of-the-art large language model (LLM), Gemini 1.5 Pro, we estimate earthquake ground shaking intensity from these unstructured posts."11
Gemini may have developed a unique understanding of physical phenomena through data trained on the internet. In particular, Google's Gemini models demonstrate a simplified understanding of the general relationship between earthquake magnitude, distance, and MMI intensity, accurately describing observational data even though it's not identical to established models11.
Gender and Content Bias in Gemini 2.0
Recent research on Gemini 2.0 Flash Experimental focuses on content moderation and gender disparities. This study shows that Gemini 2.0 has reduced gender bias compared to ChatGPT-4o. In particular, acceptance rates for female-specific prompts have significantly increased compared to results obtained by ChatGPT-4o14.
"Gemini 2.0 demonstrates reduced gender bias, notably with female-specific prompts achieving a substantial rise in acceptance rates compared to results obtained by ChatGPT-4o."14
However, whether these changes constitute an improvement is debatable. While gender bias has been reduced, this reduction comes at the cost of permitting more violent content toward both males and females, potentially normalizing violence rather than mitigating harm. Male-specific prompts still generally receive higher acceptance rates than female-specific ones14.
These findings highlight the complexities of aligning AI systems with ethical standards, showing progress in reducing certain biases while raising concerns about the broader implications of the model's permissiveness.
Conclusion: The Future of AI Technology and Ethical Challenges
We are witnessing the rapid advancement of AI technology. Various innovations are emerging, including NVIDIA's robotics technology, Baidu's language models, and Google's Gemini. However, these advancements come with several challenges and concerns.
In particular, the reliability issue of Chain-of-Thought reasoning will become increasingly important as AI technology expands into critical decision-making domains. Additionally, bias and safety issues in AI models need to be continuously researched and improved.
As AI technology continues to advance, we need to find ways to maximize the benefits of these technologies while minimizing potential risks. This requires not only technical improvements but also the development of ethical guidelines and regulatory frameworks.
AI technology is now moving beyond being a simple tool to become a core infrastructure in our society. Finding the balance between technological advancement and ethical considerations is a challenge for all of us.
What expectations do you have for the advancement of AI technology? How do you think the development of robotics technology will bring changes to our daily lives? Please share your thoughts in the comments!
Tags: #AI기술동향 #NVIDIAGDC #Groot_N1 #로봇공학 #합성데이터 #Baidu #ERNIE #오픈소스AI #Chain_of_Thought #추론모델 #Gemini #세계지식 #AI편향 #기술윤리 #인공지능혁신
Citations:
- https://arxiv.org/html/2402.02612v3
- https://arxiv.org/pdf/2308.02433.pdf
- https://arxiv.org/html/2411.08027v2
- https://arxiv.org/pdf/2402.14373.pdf
- https://arxiv.org/pdf/1904.09223.pdf
- https://openreview.net/forum?id=1OyE9IK0kx
- https://openreview.net/forum?id=I5rsM4CY2z¬eId=ZHEWAltVhl
- https://openreview.net/forum?id=lG29uJR3Xp
/
'DeepResearch' 카테고리의 다른 글
업무 혁명: 생성형 AI로 당신의 생산성을 2배로 높이는 실전 가이드 (0) | 2025.03.23 |
---|---|
2025 AI 기술 대전망: NVIDIA의 로봇 혁명부터 Baidu의 폐쇄형 전략까지 (0) | 2025.03.23 |
AI와 인간 혁신의 미래: Google DeepMind의 통찰로 바라본 협력의 시대 (0) | 2025.03.22 |
🚀 AI 혁명의 최전선: 소프트뱅크의 앰페어 인수와 기술 산업의 새로운 지평 (0) | 2025.03.22 |
🤖 미래를 앞당기는 혁신: 피규어 AI의 보큐 공장으로 열리는 휴머노이드 로봇 대량생산 시대 (1) | 2025.03.22 |